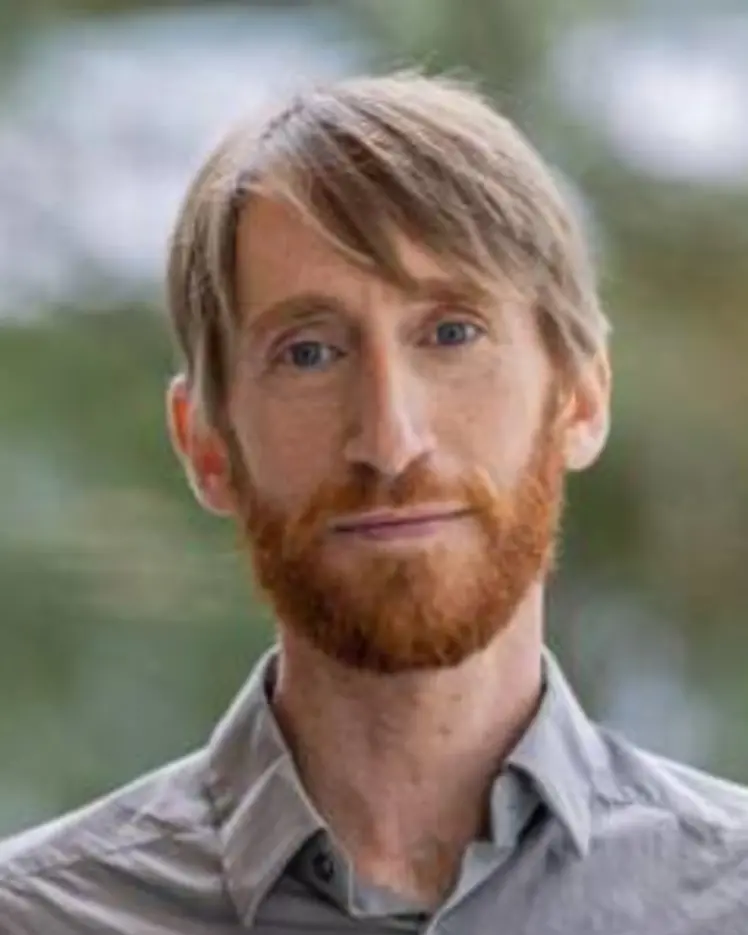
Marco Pedersoli
Biographie
Je suis professeur associé à l'ÉTS Montréal, membre du LIVIA (le Laboratoire d'Imagerie, Vision et Intelligence Artificielle), et membre du Laboratoire International des Systèmes d'Apprentissage (ILLS). Je suis également membre d'ELLIS, le réseau européen d'excellence en IA. Depuis 2021, je suis co-titulaire de la chaire de recherche industrielle Distech sur les réseaux neuronaux intégrés pour le contrôle des bâtiments connectés.
Mes recherches sont centrées sur les méthodes et algorithmes de Deep Learning, avec un accent sur la reconnaissance visuelle, l'interprétation automatique et la compréhension des images et des vidéos. L'un des principaux objectifs de mon travail est de faire progresser l'intelligence artificielle en minimisant deux facteurs critiques : la charge de calcul et la nécessité d'une supervision humaine. Ces réductions sont essentielles pour une IA évolutive, permettant des systèmes plus efficaces, adaptatifs et intégrés. Dans mes travaux récents, j'ai contribué au développement de réseaux neuronaux pour les bâtiments intelligents, en intégrant des solutions basées sur l'IA pour améliorer l'efficacité énergétique et le confort dans les environnements intelligents.