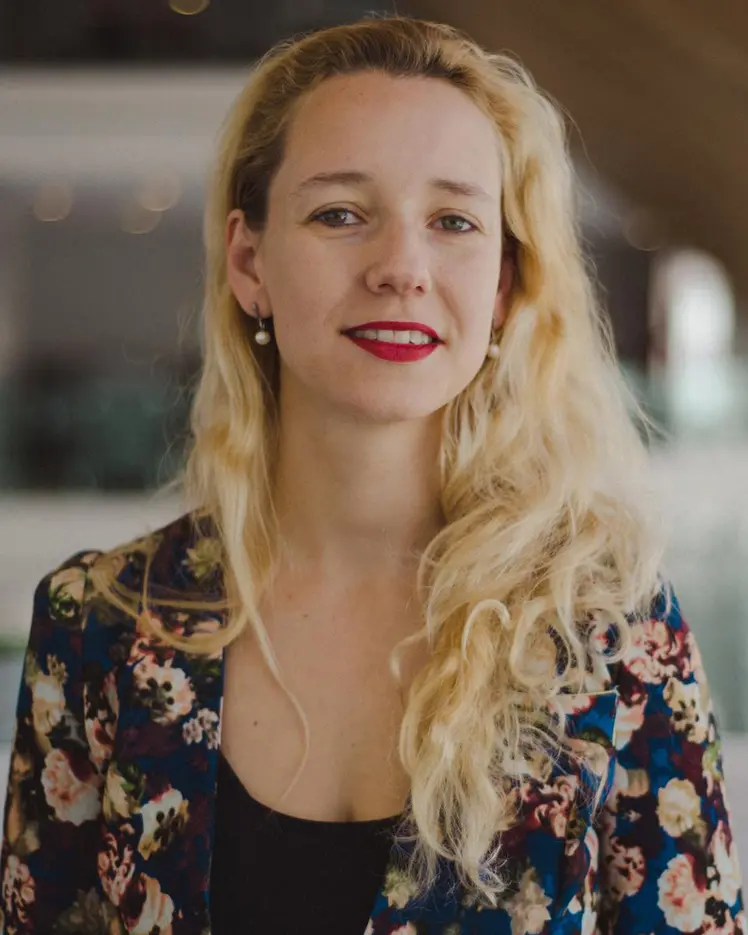
Julie Hussin
Biographie
Julie Hussin est professeure agrégée à la Faculté de médecine de l'Université de Montréal (UdeM) et chercheuse à l'Institut de cardiologie de Montréal (ICM). Elle est aussi chercheuse-boursière junior 2 du Fonds de recherche du Québec - Santé (FRQS) et responsable des programmes d'études supérieures en bio-informatique à l'UdeM.
Julie Hussin a été formée en génomique statistique et évolutive et possède une vaste expérience dans l'analyse de données multi-omiques issues de vastes cohortes populationnelles. Ses travaux en biologie computationnelle se concentrent principalement sur la génomique médicale et des populations, contribuant à plusieurs avancées méthodologiques dans ces domaines. Son travail interdisciplinaire vise à développer des outils novateurs pour la médecine de précision.
Ses projets de recherche se focalisent sur l'amélioration de la prédiction de risques et la gestion des maladies cardiométaboliques, en particulier dans le cas de l'insuffisance cardiaque. Les méthodologies utilisées dans son groupe intègrent différentes sources de données, notamment des données cliniques, génétiques, transcriptomiques, protéomiques et métabolomiques, pour permettre la découverte de nouvelles informations sur les déterminants biologiques des maladies cardiaques, notamment par des techniques d’apprentissage non supervisé. Dans le contexte de la pandémie de COVID-19, son équipe a également développé des approches d’analyse de données génétiques des virus, pour la surveillance virale et l’étude des interactions hôte-pathogène ainsi que l'évolution virale.
Ses intérêts de recherche comprennent également l'interprétabilité, la généralisation et l'équité des algorithmes d'apprentissage automatique dans la recherche en santé. Julie Hussin s'engage à promouvoir activement une IA équitable, sûre et transparente dans la recherche en santé et s'efforce d'assurer l'inclusivité et la représentativité des individus dans sa recherche, pour que son travail bénéficie à l'ensemble de la population. Elle partage son expertise en donnant plusieurs cours de bio-informatique et de génétique des populations, ainsi que d’apprentissage automatique en génomique. Avant de se joindre à l'Université de Montréal en tant que professeure, elle a été boursière postdoctorale du Human Frontier Science Program au Wellcome Trust Centre for Human Genetics de l'Université d'Oxford (Linacre College) et chercheuse postdoctorale invitée à l'Université McGill.