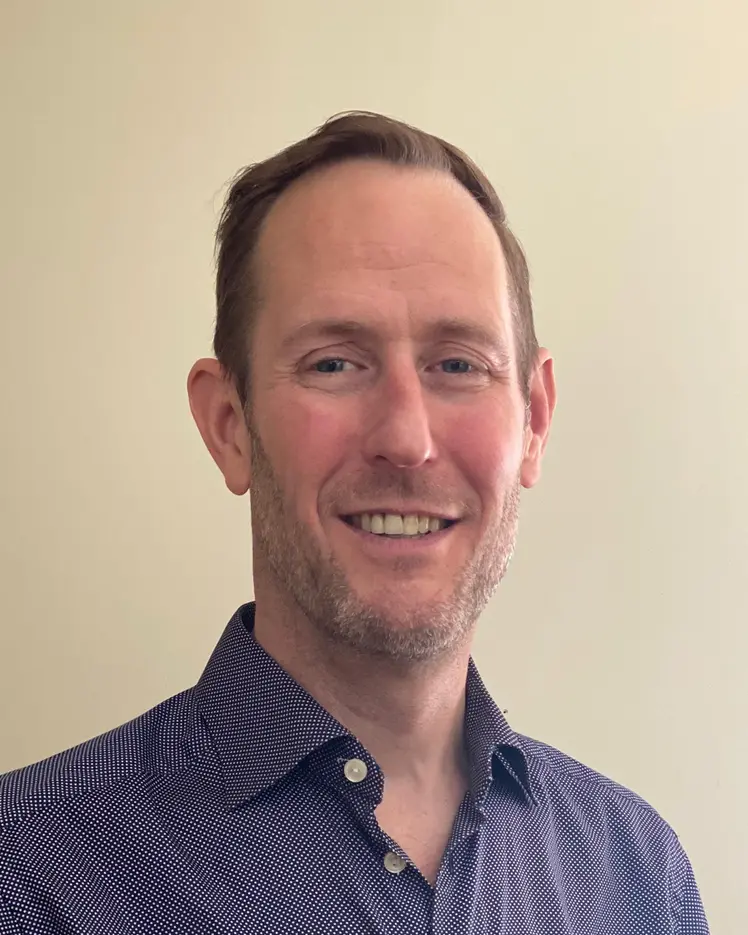
David Buckeridge
Biographie
David Buckeridge est professeur titulaire à l'École de santé des populations et de santé mondiale de l'Université McGill, responsable de la santé numérique au Centre universitaire de santé McGill et directeur scientifique exécutif pour l'Agence de la santé publique du Canada. Titulaire d'une chaire de recherche du Canada (niveau 1) en informatique de la santé et en science des données, il a établi les projections concernant la demande dans le système de santé du Québec, dirigé la gestion des données et l'analyse pour le groupe de travail sur l'immunité canadienne et aidé l'Organisation mondiale de la santé à surveiller l'immunité mondiale contre le SRAS-CoV-2. Il est titulaire d'un doctorat en médecine (Université Queen's), d'une maîtrise en épidémiologie (Université de Toronto) et d'un doctorat en informatique biomédicale (Université Stanford), et est membre du Collège royal des médecins du Canada.