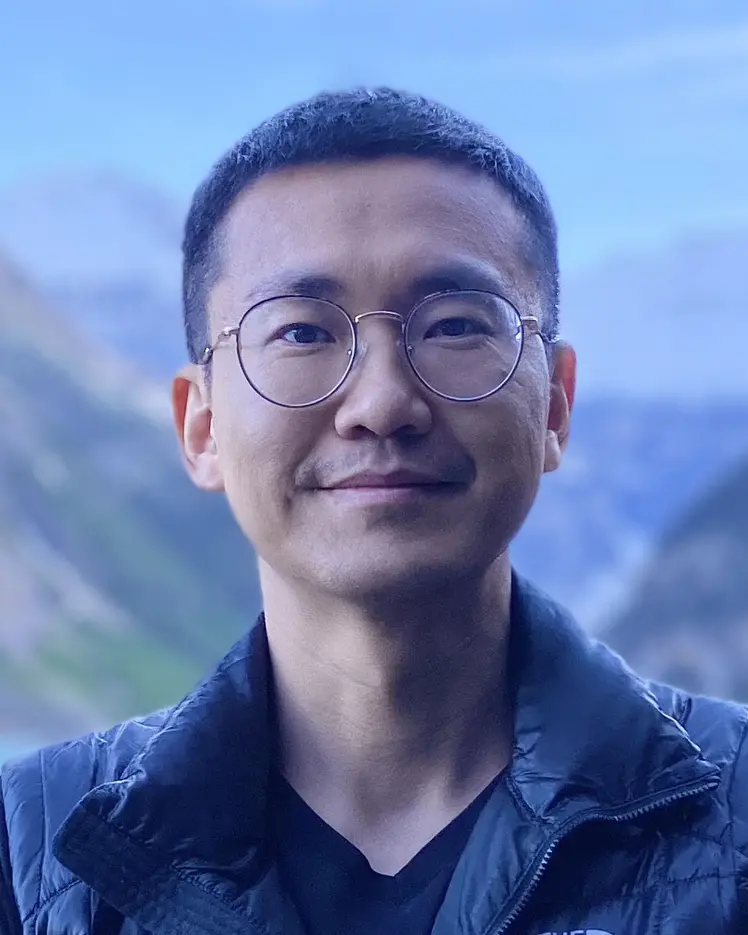
Archer Yang
Biography
Archer Yang is an associate professor in the Department of Mathematics and Statistics at McGill University, as well as an associate academic member of McGill’s School of Computer Science and the Quantitative Life Sciences program. His main research areas are statistical machine learning, statistical computing, high-dimensional statistics, causal inference and optimization, along with applications in biomedical data science.