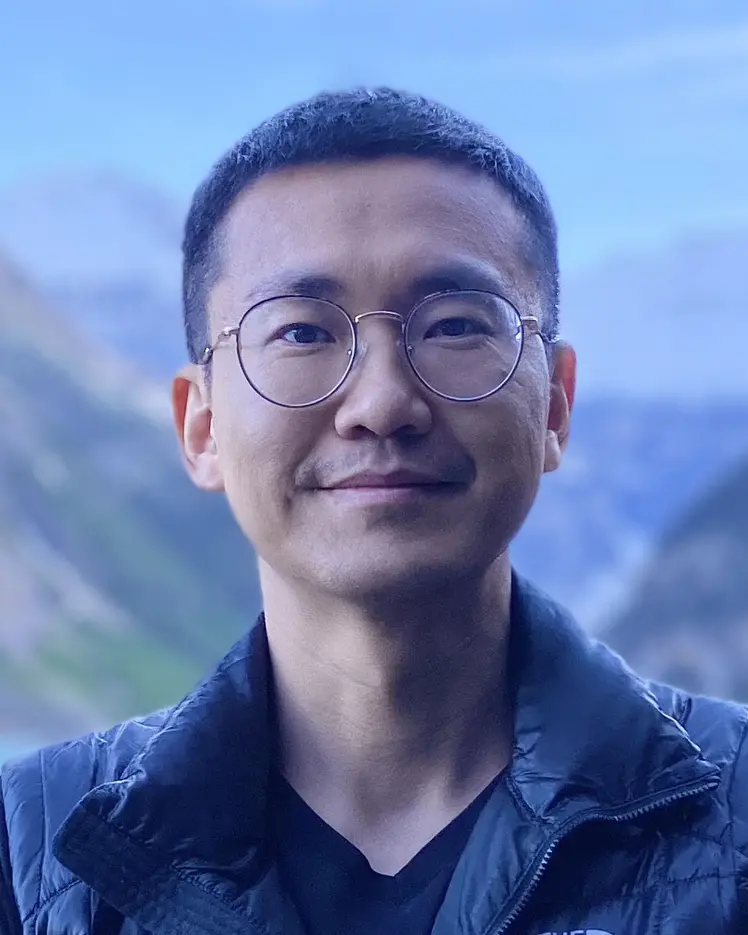
Archer Yang
Biographie
Archer Yang est professeur agrégé au Département de mathématiques et de statistiques de l'Université McGill et membre associé de l'École d'informatique et du programme des Sciences quantitatives de la vie. Ses principaux domaines de recherche sont l'apprentissage automatique statistique, l'informatique statistique, les statistiques à haute dimension, l'inférence causale et l'optimisation avec des applications en science des données biomédicales.