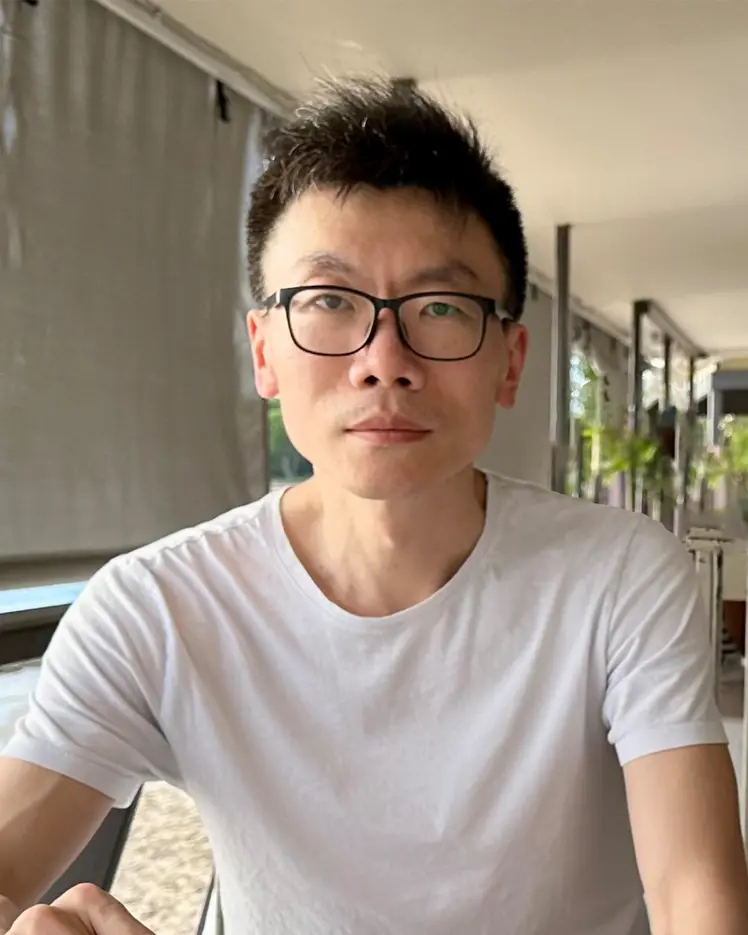
Biographie
J'ai obtenu un doctorat en informatique et biologie computationnelle de l'Université de Toronto en 2014. Avant de me joindre à l’Université McGill, j'ai été associé postdoctoral au Computer Science and Artificial Intelligence Laboratory (CSAIL) du Massachusetts Institute of Technology (MIT) (2015-2018).
Mes recherches portent sur le développement de modèles d'apprentissage probabilistes interprétables et de modèles d'apprentissage profond pour modéliser les données génétiques et épigénétiques, les dossiers de santé électroniques et les données génomiques unicellulaires.
En intégrant systématiquement des données multimodales et longitudinales, je cherche à obtenir des applications qui auront des effets tangibles en médecine computationnelle, y compris la construction de systèmes de recommandation clinique intelligents, la prévision des trajectoires de santé des patients, les prédictions personnalisées de risques polygéniques, la caractérisation des mutations génétiques fonctionnelles multitraits, et la dissection des éléments réglementaires spécifiques au type de cellule qui sont à la base des traits complexes et des maladies chez l'homme. Mon programme de recherche couvre trois domaines principaux impliquant l'apprentissage automatique appliqué à la génomique computationnelle et à la santé.