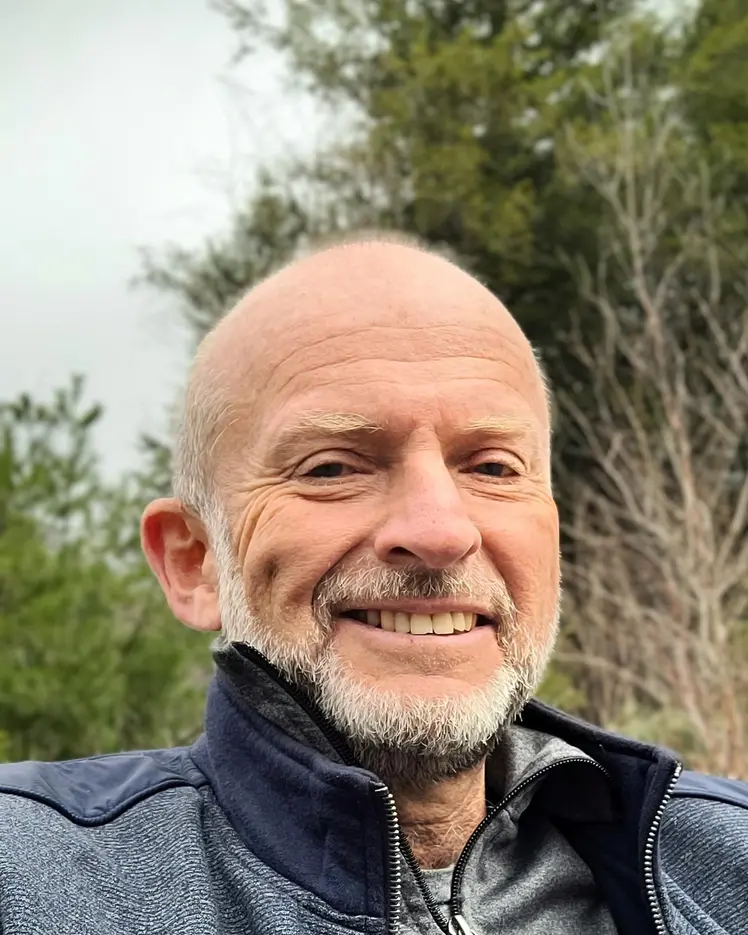
Dan Poenaru
Biographie
Dan Poenaru est professeur de chirurgie pédiatrique à l’Université McGill et chercheur principal à l’Institut de recherche du Centre universitaire de santé McGill, à Montréal. Il est titulaire d’une maîtrise en éducation aux professions de la santé et en développement international, et d’un doctorat en stratégie et gestion de la santé.
Chercheur financé par le Fonds de recherche du Québec - Santé (FRQS) et les Instituts de recherche en santé du Canada (IRSC) dans le domaine des soins chirurgicaux centrés sur le patient, il est également chef du laboratoire CommiSur de l’Université McGill, directeur de la bourse Jean-Martin-Laberge en chirurgie pédiatrique mondiale et membre fondateur de l’Initiative mondiale pour la chirurgie infantile (GICS).
Ses domaines d’intérêt actuels sont la communication chirurgicale et l’enseignement médical assistés par la technologie, y compris l’IA, la réalité virtuelle et les dispositifs de santé numériques, les soins chirurgicaux centrés sur le patient et le développement de la capacité de recherche chirurgicale mondiale.