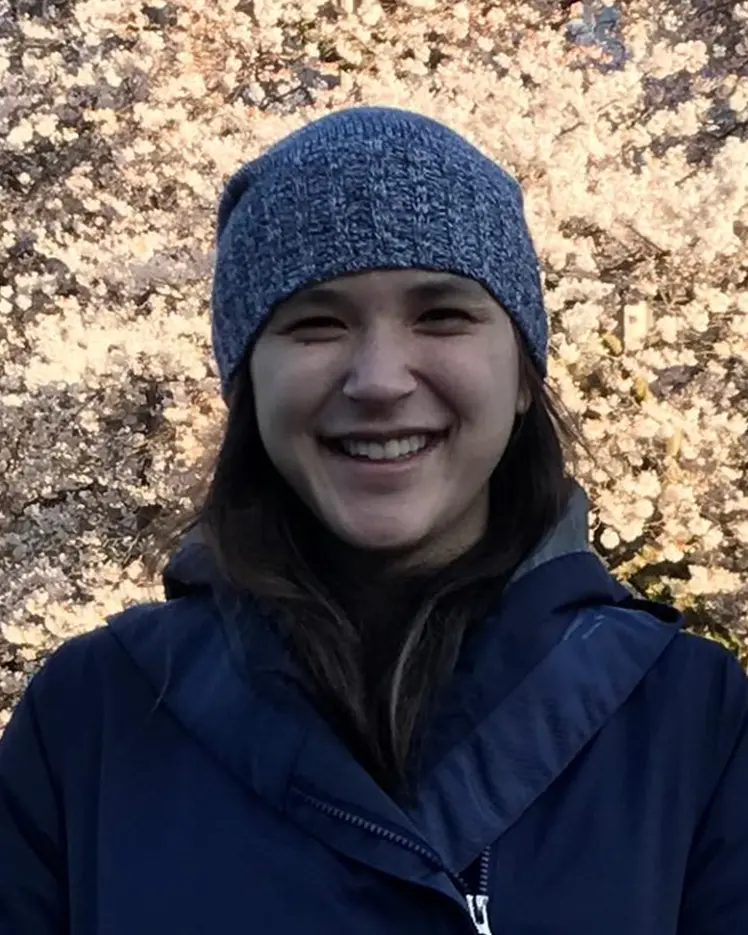
Courtney Paquette
Biographie
Courtney Paquette est professeure adjointe à l'Université McGill et titulaire d'une chaire en IA Canada-CIFAR à Mila – Institut québécois d’intelligence artificielle. Sa recherche se concentre sur la conception et l'analyse d'algorithmes pour les problèmes d'optimisation à grande échelle, et vise des applications en science des données. Courtney Paquette a obtenu un doctorat en mathématiques de l'Université de Washington (2017), a occupé des postes postdoctoraux à l'Université Lehigh (2017-2018) et à l'Université de Waterloo (bourse postdoctorale de la NSF, 2018-2019), et a été chercheuse scientifique chez Google Research, Brain Montréal (2019-2020).