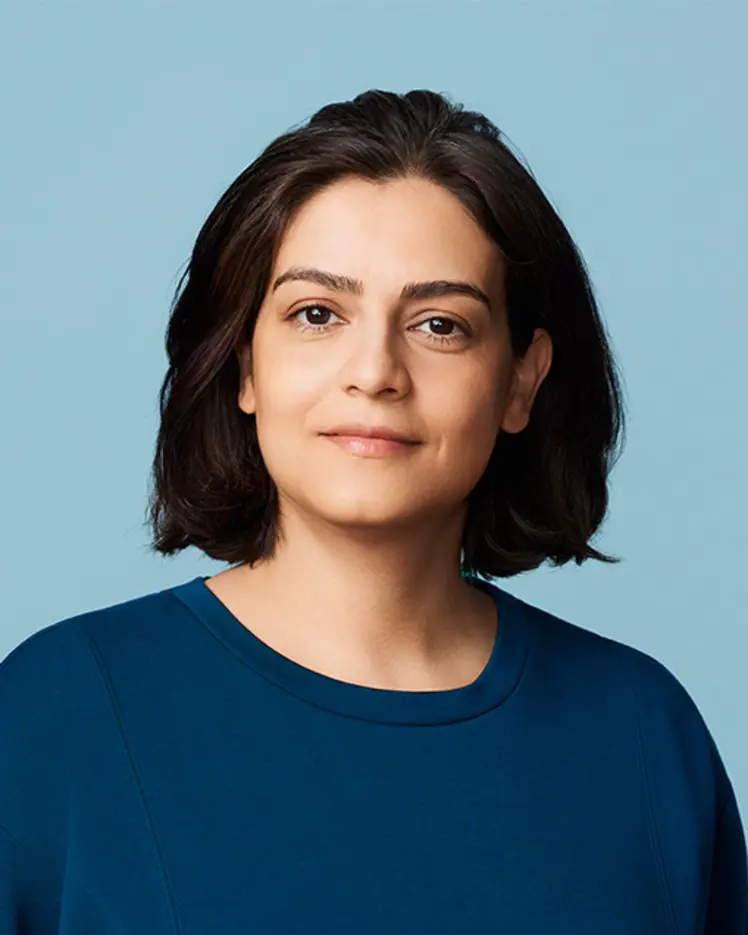
Reihaneh Rabbany
Biography
Reihaneh Rabbany is an assistant professor at the School of Computer Science, McGill University, and a core academic member of Mila – Quebec Artificial Intelligence Institute. She is also a Canada CIFAR AI Chair and on the faculty of McGill’s Centre for the Study of Democratic Citizenship.
Before joining McGill, Rabbany was a postdoctoral fellow at the School of Computer Science, Carnegie Mellon University. She completed her PhD in the Department of Computing Science at the University of Alberta.
Rabbany heads McGill’s Complex Data Lab, where she conducts research at the intersection of network science, data mining and machine learning, with a focus on analyzing real-world interconnected data and social good applications.