Accueil
Inspirer le développement de l'intelligence artificielle au bénéfice de tous·tes
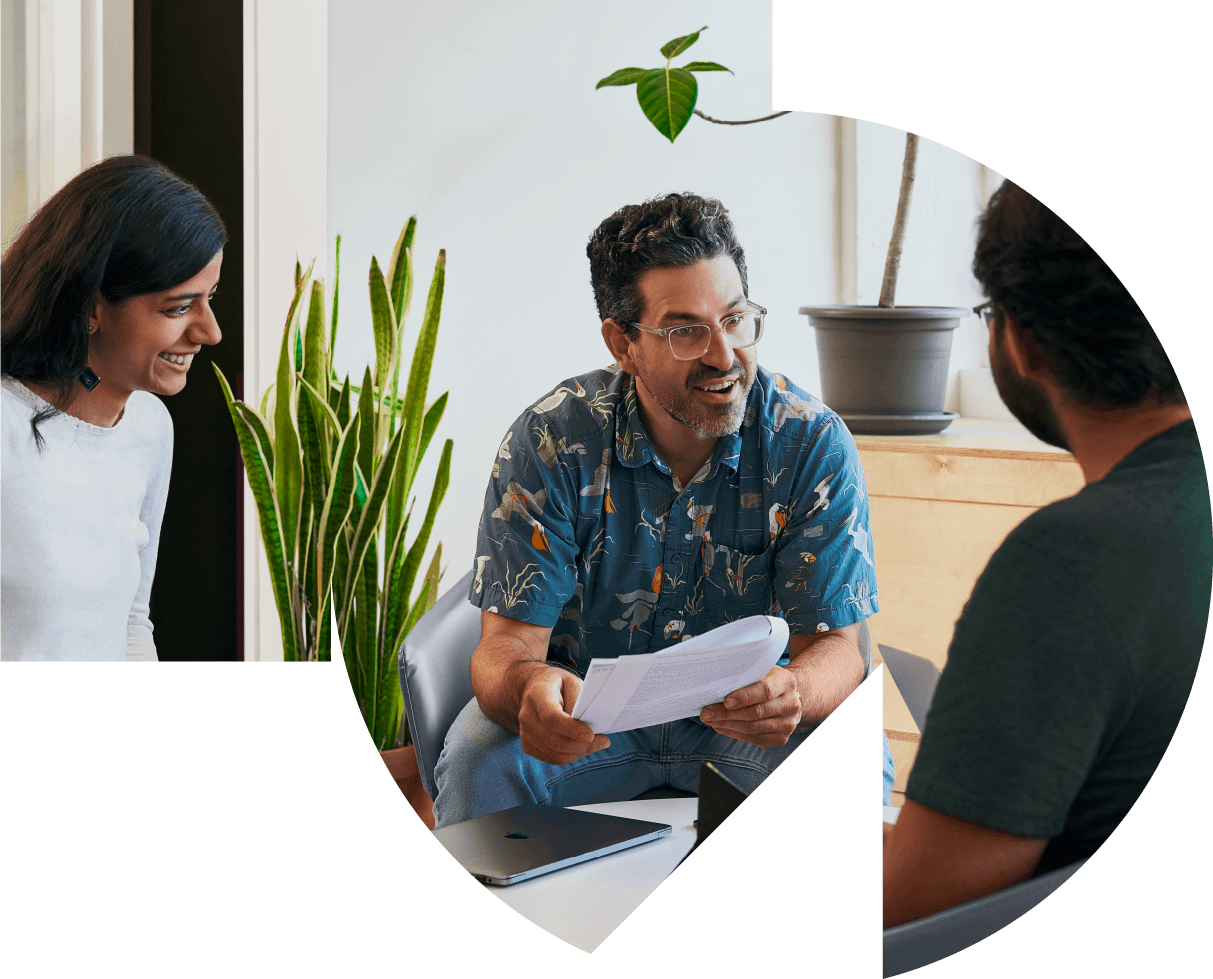
Fondé en 1993 par le professeur Yoshua Bengio, Mila regroupe aujourd'hui plus de 140 professeur·e·s affilié·e·s à l'Université de Montréal, l'Université McGill, Polytechnique Montréal et HEC Montréal. L'institut accueille également des professeur·e·s de l'Université Laval, de l'Université de Sherbrooke, de l'École de technologie supérieure (ÉTS) et de l'Université Concordia.
Le développement socialement responsable et bénéfique de l'IA est une dimension fondamentale de la mission de Mila. En tant que chef de file, nous souhaitons contribuer au dialogue social et au développement d'applications qui seront bénéfiques pour la société.