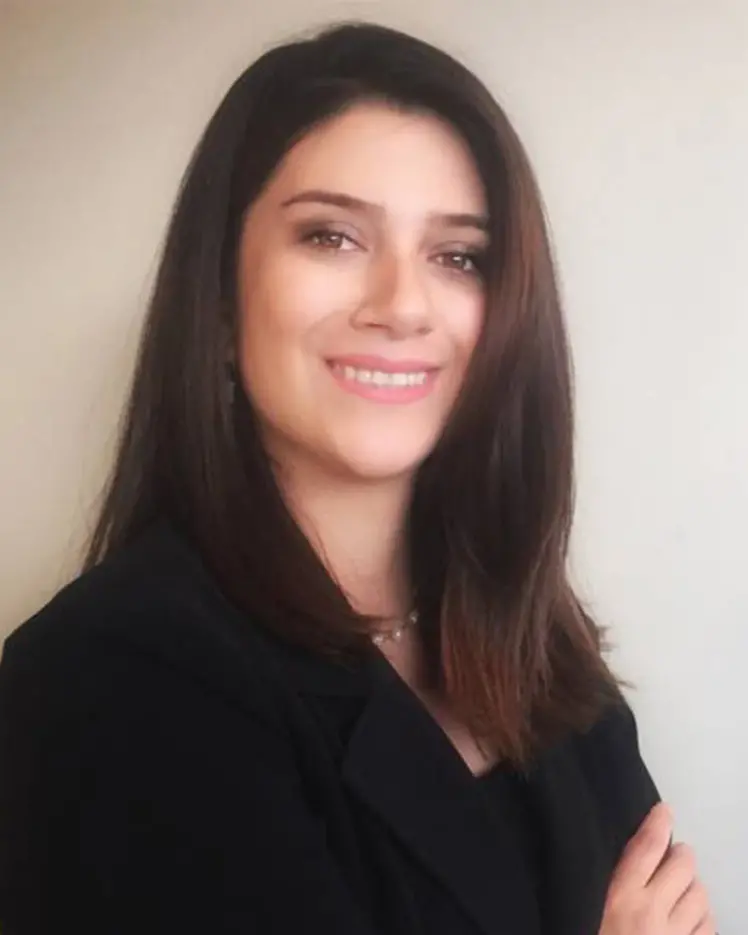
Samira Abbasgholizadeh-Rahimi
Biographie
Samira Abbasgholizadeh-Rahimi (B. Ing., Ph. D.) est titulaire de la Chaire de recherche du Canada sur les soins de santé primaires numériques avancés et professeure adjointe au Département de médecine familiale de l'Université McGill et à Mila – Institut québécois d'intelligence artificielle.
Elle est également scientifique affiliée à l'Institut Lady Davis de recherches médicales de l'Hôpital général juif, présidente élue de la Société canadienne de recherche opérationnelle et directrice d'Intelligence artificielle en médecine familiale (AIFM).
Bénéficiant de sa formation interdisciplinaire, ses travaux portent sur le développement et la mise en œuvre de technologies de santé numérique avancées, telles que les outils d'aide à la décision basés sur l'IA, dans les soins de santé primaires. Ses recherches sont consacrées à l'amélioration de la prévention et de la gestion des maladies chroniques, dont les maladies cardiovasculaires, avec un accent particulier sur les populations vulnérables.
Les travaux qu’elle a menés en tant que chercheuse principale ont été financés par le Fonds de recherche du Québec - Santé (FRQS), le Conseil de recherches en sciences naturelles et en génie (CRSNG), Roche Canada, la Fondation Brocher (Suisse) et la Stratégie de recherche axée sur le patient (SRAP) - Instituts de recherche en santé du Canada (IRSC).
Elle a reçu de nombreux prix, notamment le prix New Investigator Primary Care Research 2022 du North American Primary Care Research Group (NAPCRG), qui récompense les contributions exceptionnelles de nouveaux chercheurs dans le domaine de la recherche sur les soins primaires.