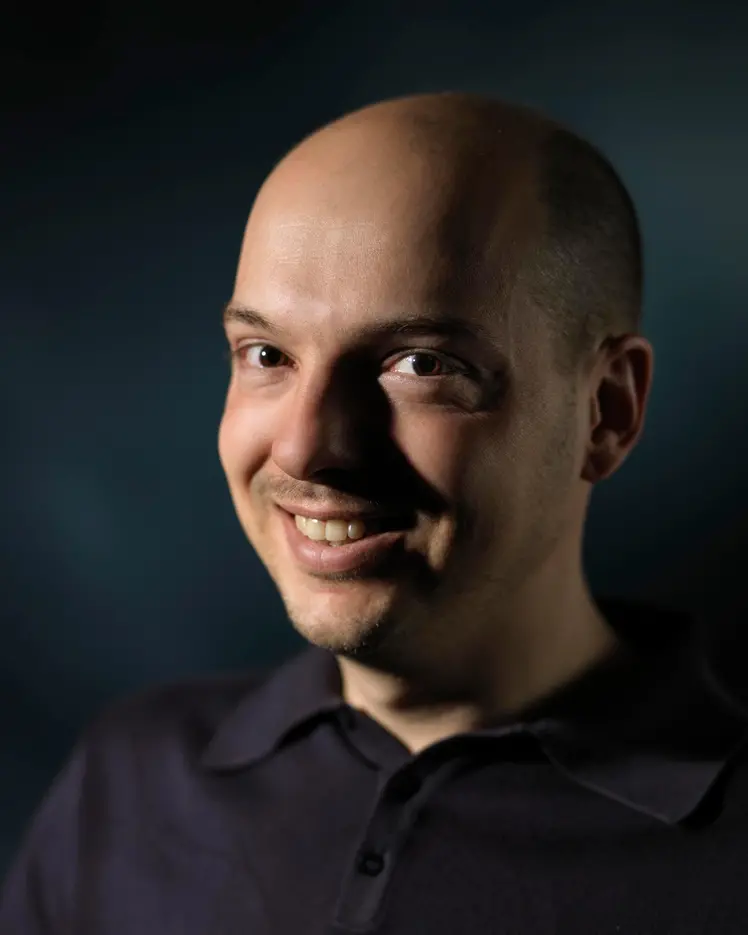
Pablo Piantanida
Biographie
Je suis professeur au CentraleSupélec de l'Université Paris-Saclay avec le Centre national français de la recherche scientifique (CNRS), et directeur du Laboratoire international sur les systèmes d'apprentissage (ILLS) qui regroupe l'Université McGill, l'École de technologie supérieure (ÉTS), Mila - Institut québécois d'intelligence artificielle, le Centre national français de la recherche scientifique (CNRS), l'Université Paris-Saclay et l'École CentraleSupélec.
Mes recherches portent sur l'application de techniques statistiques et de théorie de l'information avancées au domaine de l'apprentissage automatique. Je m'intéresse au développement de techniques rigoureuses basées sur des mesures et des concepts d'information pour construire des systèmes d'IA sûrs et fiables et établir la confiance dans leur comportement et leur robustesse, sécurisant ainsi leur utilisation dans la société. Mes principaux domaines d'expertise sont la théorie de l'information, la géométrie de l'information, la théorie de l'apprentissage, la protection de la vie privée, l'équité, avec des applications à la vision par ordinateur et au traitement du langage naturel.
J'ai fait mes études de premier cycle à l'université de Buenos Aires et j'ai poursuivi des études supérieures en mathématiques appliquées à l'université Paris-Saclay en France. Tout au long de ma carrière, j'ai également occupé des postes d'invité à l'INRIA, à l'Université de Montréal et à l'École de technologie supérieure (ÉTS), entre autres.
Mes recherches antérieures ont porté sur les domaines de la théorie de l'information au-delà de la compression distribuée, de la décision statistique, du codage universel des sources, de la coopération, de la rétroaction, du codage d'index, de la génération de clés, de la sécurité et de la protection des données.
Je donne des cours sur l'apprentissage automatique, la théorie de l'information et l'apprentissage profond, couvrant des sujets tels que la théorie de l'apprentissage statistique, les mesures de l'information, les principes statistiques des réseaux neuronaux.