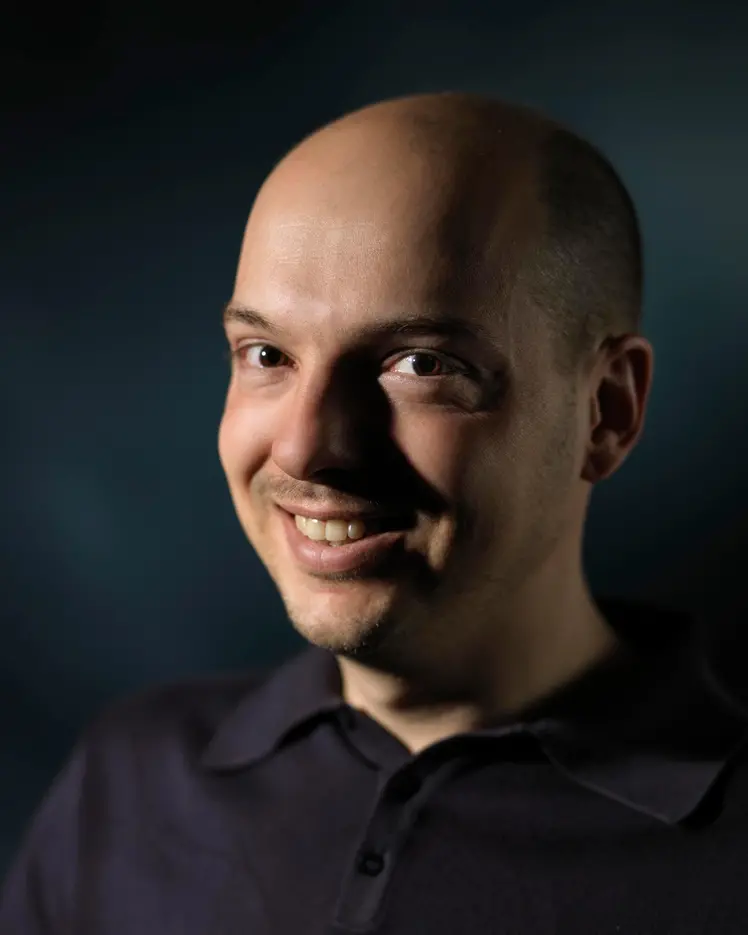
Pablo Piantanida
Biography
I am a professor at CentraleSupélec (Université Paris-Saclay) with the French National Centre for Scientific Research (CNRS), and Director of the International Laboratory on Learning Systems (ILLS) which gathers McGill University, École de technologie supérieure (ÉTS), Mila – Quebec AI Institute, France’s Centre Nationale de la Recherche Scientifique (CNRS), Université Paris-Saclay, and the École CentraleSupélec.
My research revolves around the application of advanced statistical and information-theoretic techniques to the field of machine learning. I am interested in developing rigorous techniques based on information measures and concepts for building safe and trustworthy AI systems and establishing confidence in their behavior and robustness, thereby securing their use in society. My primary areas of expertise include information theory, information geometry, learning theory, privacy, fairness, with applications to computer vision and natural language processing.
I obtained my undergraduate education at the University of Buenos Aires and pursued graduate studies in applied mathematics at Paris-Saclay University in France. Throughout my career, I have also held visiting positions at INRIA, Université de Montréal and Ecole de Technologie Supérieure (ÉTS), among others.
My earlier research encompassed the fields of information theory beyond distributed compression, statistical decision, universal source coding, cooperation, feedback, index coding, key generation, security, and privacy, among others.
I teach courses on machine learning, information theory and deep learning, covering topics such as statistical learning theory, information measures, statistical principles of neural networks.