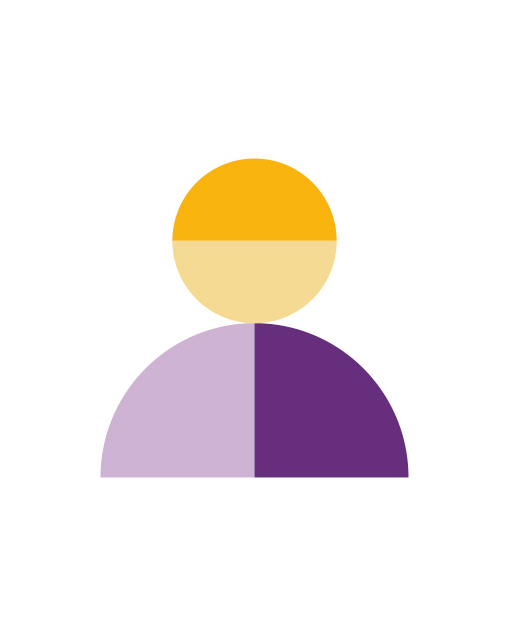
Michael Rabbat
Biographie
Mike Rabbat est membre affilié de Mila – Institut québécois d’intelligence artificielle et directeur de la recherche scientifique au sein de l'équipe FAIR (Fundamental AI Research) de Meta. Ses recherches portent sur l'apprentissage efficace et robuste des représentations, en particulier l'apprentissage autosupervisé. Il s'intéresse également à l'optimisation pour un apprentissage efficace des modèles.