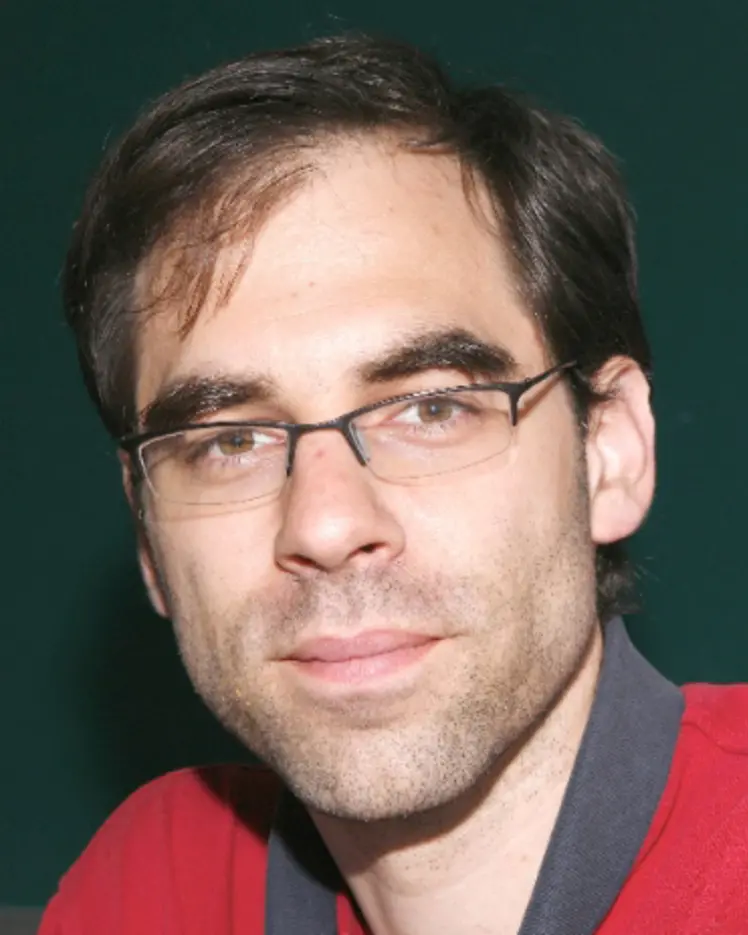
Mathieu Blanchette
Biographie
Mathieu Blanchette est professeur associé et directeur de l'École d'informatique de l'Université McGill.
Après avoir obtenu un doctorat (Université de Washington, 2002) et un postdoctorat (Université de Californie à Santa Cruz, 2003), il s'est joint à l'École d'informatique de l’Université McGill et a fondé le Laboratoire de génomique computationnelle. Les recherches effectuées par son équipe d’exception ont fait l'objet de plus de 70 publications. Récemment élu membre du Collège de nouveaux chercheurs et créateurs en art et science de la Société royale du Canada, il a été boursier Sloan (2009) et a reçu le prix Outstanding Young Computer Scientist Researcher de l'Association canadienne de l'informatique (2012) ainsi que le prix Chris Overton (2006). Il adore enseigner et superviser les étudiant·e·s, et a d’ailleurs reçu le prix Leo Yaffe pour l'enseignement (2008).