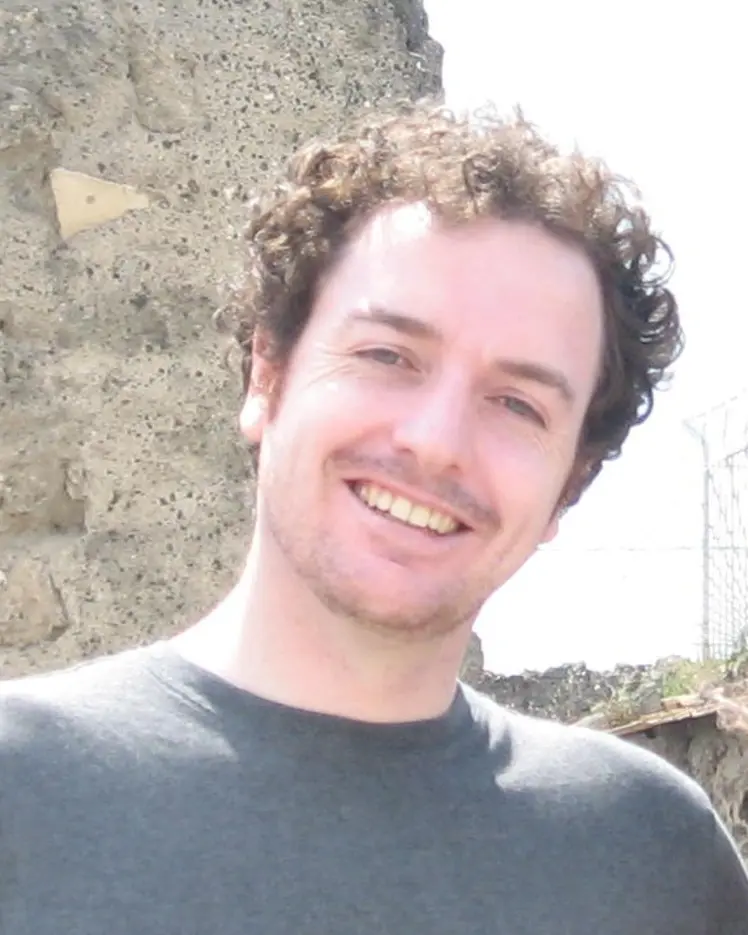
Mark Coates
Biographie
Mark Coates est professeur au Département de génie électrique et informatique de l'Université McGill, auquel il s’est joint en 2002. Il a obtenu une licence en génie des systèmes informatiques de l'Université d'Adélaïde (Australie) en 1995 et un doctorat en génie de l'information de l'Université de Cambridge (Royaume-Uni) en 1999. Il a été associé de recherche et conférencier à l'Université Rice, au Texas, de 1999 à 2001. En 2012-2013, il a travaillé en tant que scientifique principal chez Winton Capital Management à Oxford, au Royaume-Uni. Il a assumé de multiples rôles éditoriaux, notamment en tant que rédacteur principal pour IEEE Signal Processing Letters, rédacteur associé pour IEEE Transactions on Signal Processing et rédacteur associé pour IEEE Transactions on Signal and Information Processing over Networks. Les recherches de Mark Coates portent sur l'apprentissage automatique et le traitement statistique des signaux, l'inférence bayésienne et Monte Carlo, et l'apprentissage sur les graphes et les réseaux. Ses contributions les plus influentes et les plus citées concernent la tomographie des réseaux et le filtrage distribué des particules.