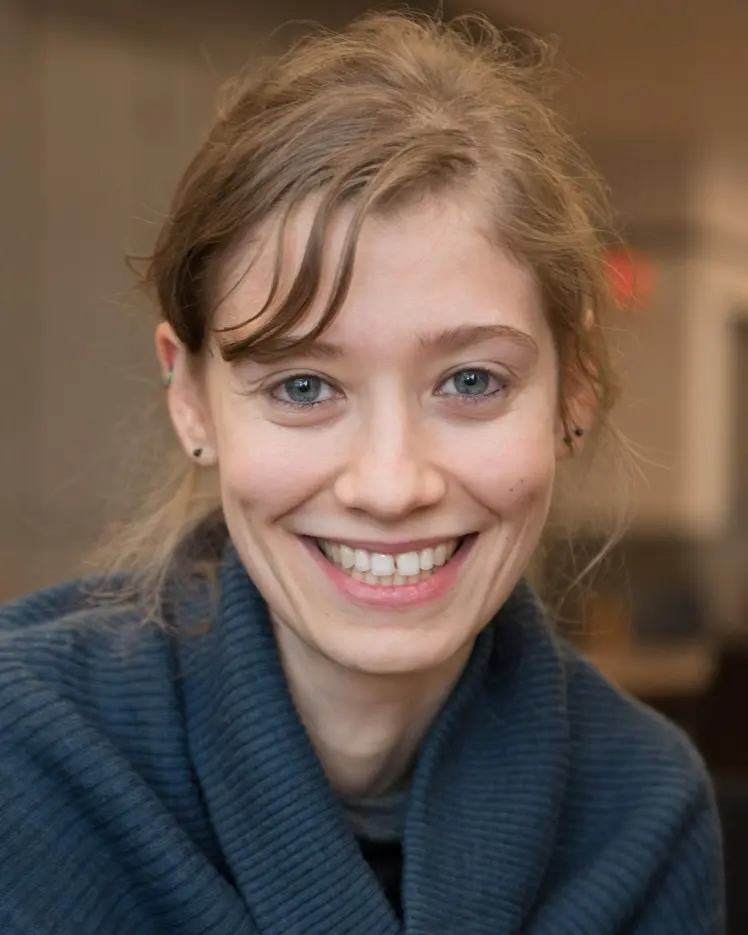
Margarida Carvalho
Biographie
Margarida Carvalho est titulaire d'un baccalauréat et d'une maîtrise en mathématiques. Elle a obtenu un doctorat en informatique à l'Université de Porto, pour lequel elle a reçu le prix de la thèse EURO en 2018. La même année, elle est devenue professeure adjointe au Département d'informatique et de recherche opérationnelle de l'Université de Montréal, où elle occupe la Chaire de recherche FRQ-IVADO en science des données pour la théorie des jeux combinatoires.
Elle est une experte en recherche opérationnelle, notamment en optimisation combinatoire et en théorie algorithmique des jeux. Ses recherches sont motivées par des problèmes de prise de décision du monde réel impliquant l'interaction de plusieurs agents, tels que les programmes d'échange de reins, le choix des écoles et les marchés concurrentiels.