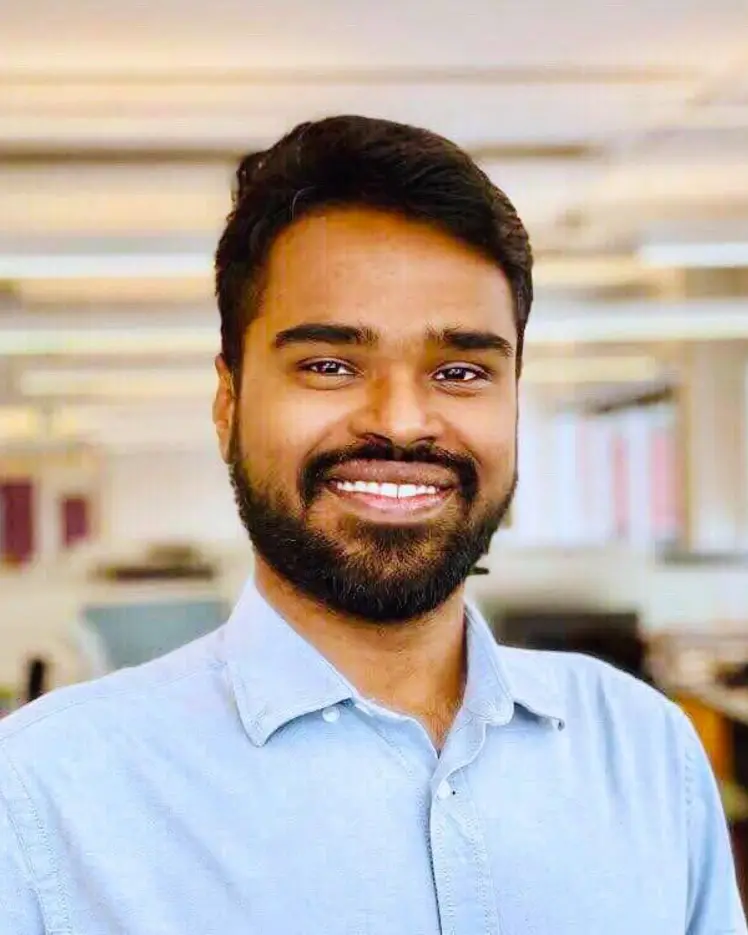
Sarath Chandar
Biography
Sarath Chandar is an associate professor at Polytechnique Montreal's Department of Computer and Software Engineering, where he leads the Chandar Research Lab. He is also a Core Academic Member at Mila – Quebec Artificial Intelligence Institute and holds a Canada CIFAR AI Chair and the Canada Research Chair in Lifelong Machine Learning.
Chandar’s research interests include lifelong learning, deep learning, optimization, reinforcement learning and natural language processing. To promote research in lifelong learning, Chandar created the Conference on Lifelong Learning Agents (CoLLAs) in 2022, for which he served as program chair in 2022 and 2023.
He has a PhD from Université de Montréal and an MSc (By Research) from the Indian Institute of Technology Madras.