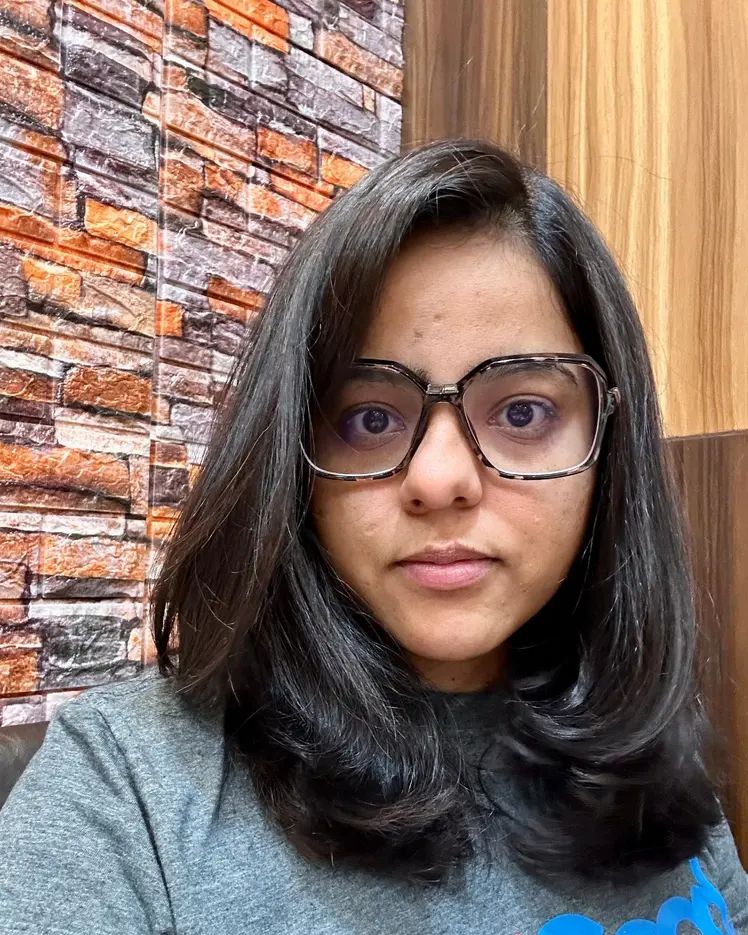
Khimya Khetarpal
Biography
Khimya Khetarpal is a Research Scientist at Google Deepmind. She earned her PhD in Computer Science from the Reasoning and Learning Lab at McGill University and Mila, advised by Doina Precup. She is broadly interested in artificial intelligence and reinforcement learning. Her current research interests focus on how RL agents learn to efficiently represent the world's knowledge, plan with it, and adapt to changes over time. Khimya’s work has appeared in leading AI journals and conferences including NeurIPS, ICML, AAAI, AISTATS, ICLR, The Knowledge Engineering Review, ACM, JAIR and TMLR. Her work has also been featured in MIT Technology Review. She was recognized as a TMLR expert reviewer in 2023, one of the Rising Stars in EECS 2020, a finalist for Three Minute Thesis (3MT) competition in AAAI 2019, selected for the Doctoral Consortium at AAAI 2019, and awarded Best Paper Award (3rd Price) for an ICML 2018 workshop on lifelong learning. Throughout her career, she has sought to actively mentor through initiatives such as co-founding the Mila peer advising initiative, teaching and assisting AI4Good Lab, volunteering at Skype A Scientist, and mentoring at FIRST Robotics.
Her research aims to (1) understand intelligent behavior that bridges both action and perception grounded in theoretical foundations of reinforcement learning, and (2) build AI agents to efficiently represent the world's knowledge, plan with it, and adapt to changes over time through learning and interaction.
She currently approaches this with the following research directions:
- Selective Attention for Fast Adaptation and Robustness
- Learning Abstractions and Affordances
- Discovery and Continual Reinforcement Learning