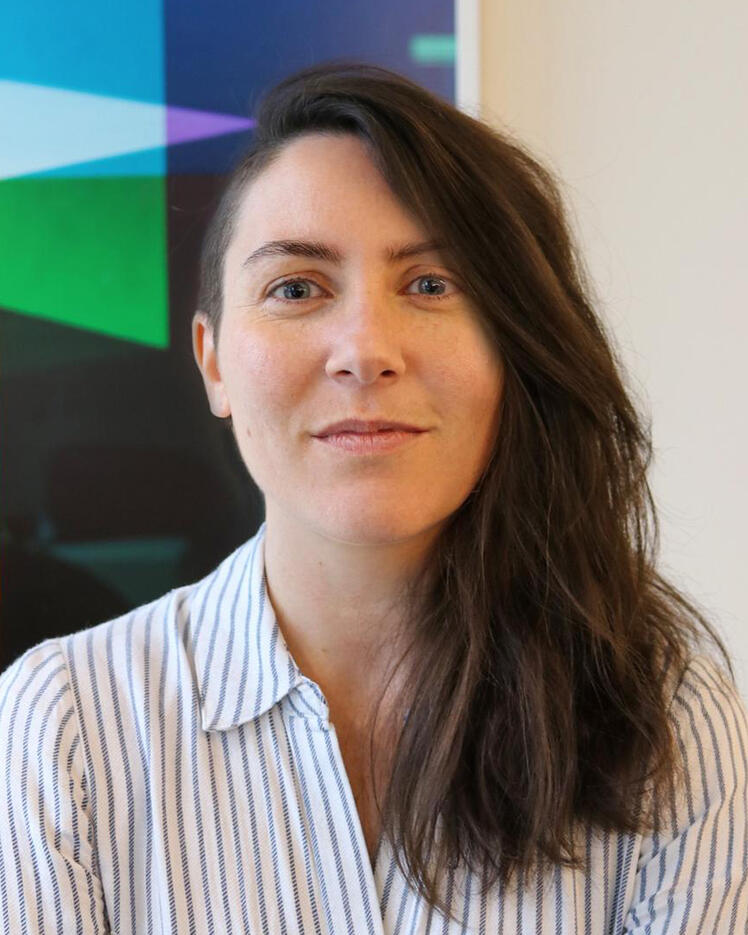
Audrey Durand
Biographie
Audrey Durand est professeure adjointe au Département d’informatique et de génie logiciel ainsi qu’au Département de génie électrique et de génie informatique de l’Université Laval. Elle se spécialise dans les algorithmes qui apprennent par l’interaction avec leur environnement, soit l’apprentissage par renforcement, et s’intéresse particulièrement à l’application de ces approches au domaine de la santé.