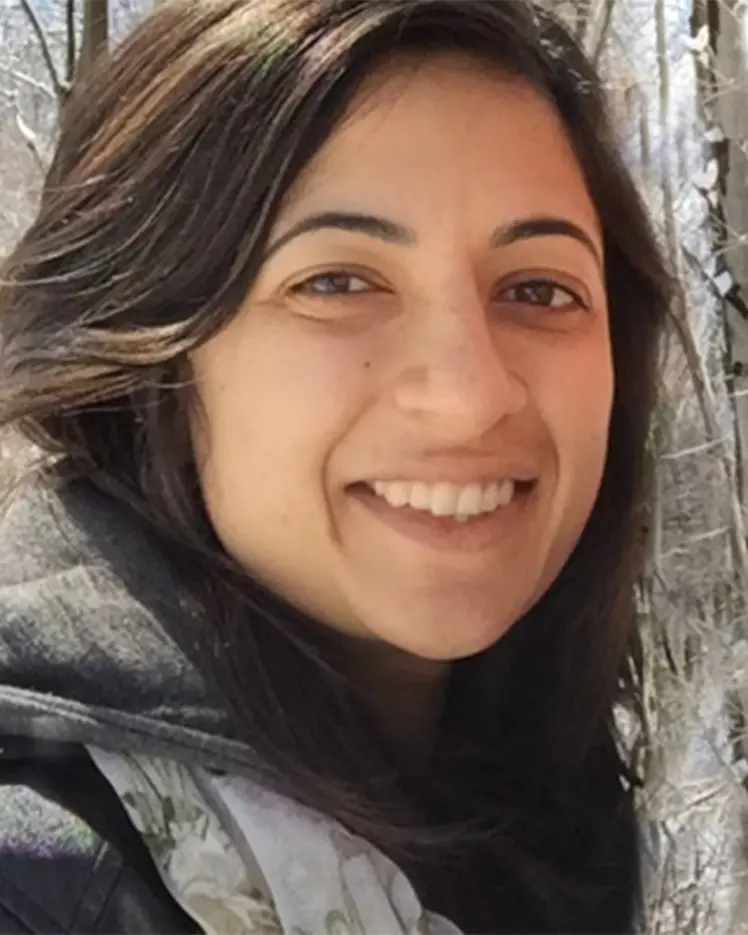
Biographie
Je suis professeur adjoint à la Faculté de l’information de l’Université de Toronto.
Mes objectifs de recherche sont de contribuer à la compréhension et aux techniques de la science du développement responsable de l’IA, tout en appliquant utilement l’IA à des problèmes écologiques à fort impact liés au changement climatique, à l’épidémiologie, à l’alignement de l’IA et à l’évaluation des impacts écologiques. Mes travaux récents portent sur deux thèmes : l’utilisation de modèles profonds pour l’analyse des politiques et l’atténuation des risques; et la conception de données ou d’environnements de tests unitaires pour évaluer empiriquement le comportement d’apprentissage ou simuler le déploiement d’un système d’IA. N’hésitez pas à me contacter pour toute collaboration dans ces domaines.
Je suis généralement intéressé par l’étude de ce qui « entre » dans les modèles profonds : non seulement les données, mais l’environnement d’apprentissage plus globalement, qui comprend la conception/spécification des tâches, la fonction de perte et la régularisation, ainsi que le contexte sociétal du déploiement, notamment les considérations de confidentialité, les tendances et les incitatifs, les normes et les préjugés humains. Je suis préoccupé et passionné par l’éthique de l’IA, la sécurité et l’application de l’apprentissage machine à la gestion de l’environnement, à la santé et au bien-être social.