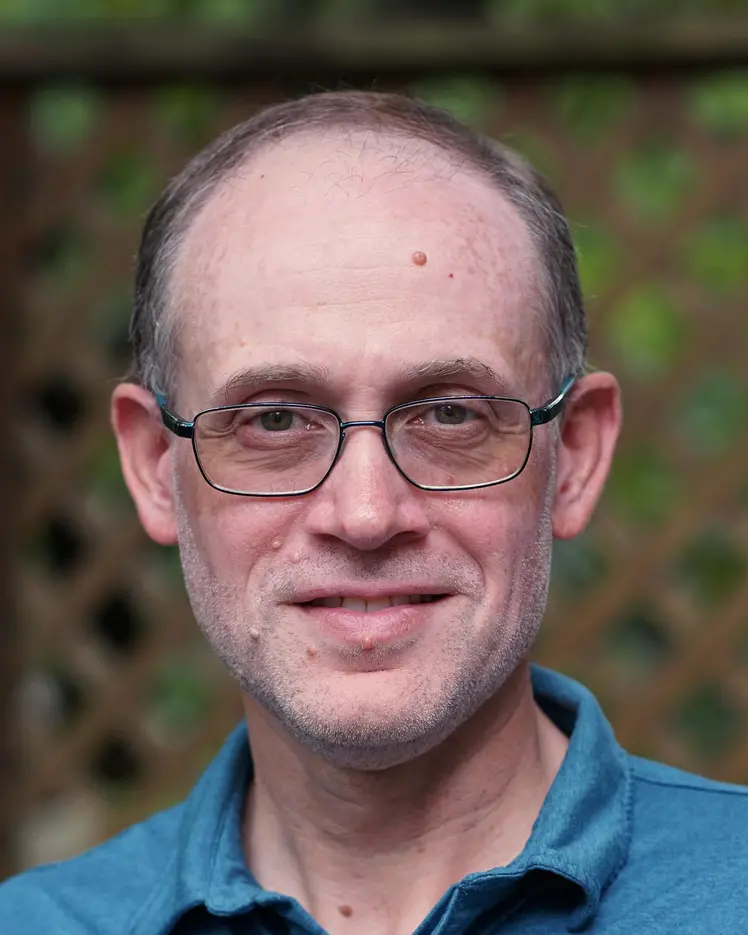
Geoff Gordon
Biographie
Geoffrey Gordon est professeur au Département d'apprentissage automatique de l'Université Carnegie Mellon, où il a également occupé les postes de directeur de département par intérim et de directeur de département associé à l'éducation.
Ses recherches ont porté sur les systèmes d’intelligence artificielle capables de penser à long terme, notamment afin de réfléchir à l’avance pour résoudre un problème, de planifier une séquence d’actions ou de déduire des propriétés invisibles à partir d’observations. Plus particulièrement, il explore la façon de combiner l'apprentissage automatique avec ces tâches de réflexion à long terme.
Geoffrey Gordon a obtenu un baccalauréat en informatique de l’Université Cornell en 1991 et un doctorat en informatique de l’Université Carnegie Mellon en 1999. Ses intérêts de recherche comprennent l’intelligence artificielle, l’apprentissage statistique, les données pédagogiques, la théorie des jeux, les systèmes multirobots et les domaines à somme générale. Auparavant, il a été professeur invité au Département de science informatique de l’Université Stanford et scientifique principal à Burning Glass Technologies, à San Diego.