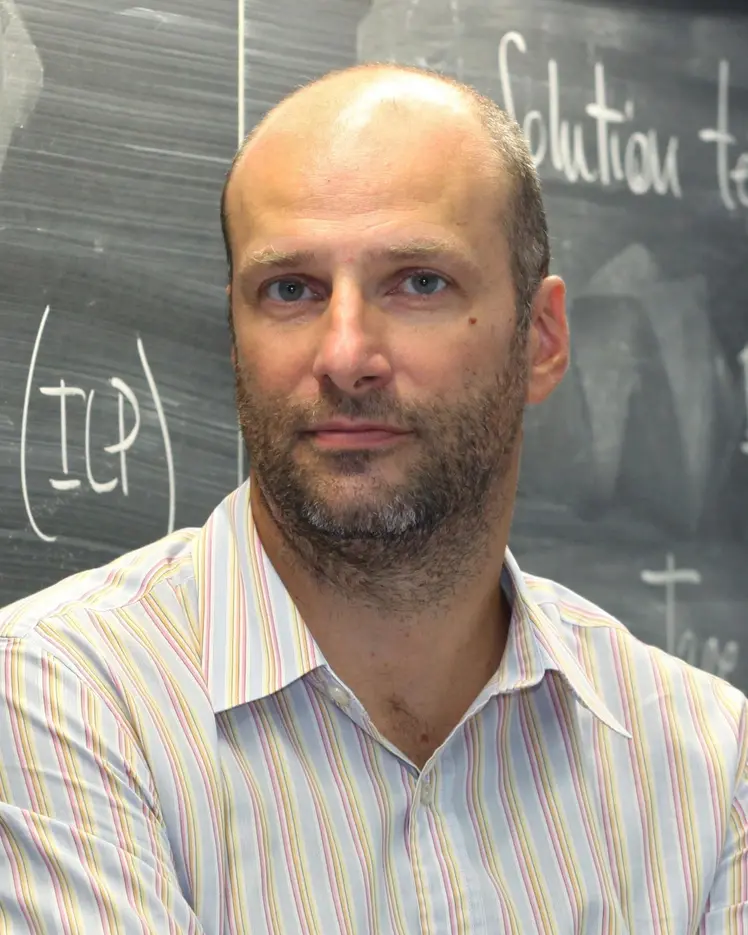
Andrea Lodi
Biographie
Andrea Lodi est professeur associé au Département de mathématiques et de génie industriel de Polytechnique Montréal. Il est aussi le fondateur et directeur scientifique d’IVADO Labs.
Depuis 2014, il est titulaire de la Chaire d'excellence en recherche du Canada sur la science des données pour la prise de décision en temps réel (Polytechnique Montréal), la chaire de recherche la plus importante au pays dans le domaine de la recherche opérationnelle. Reconnu internationalement pour ses travaux sur la programmation mixte linéaire et non linéaire, le professeur Lodi se concentre sur le développement de nouveaux modèles et algorithmes permettant de traiter rapidement et efficacement de vastes quantités de données de multiples sources. Ces algorithmes et modèles devraient conduire à la création de stratégies optimisées de prise de décision en temps réel. La Chaire a pour objectif d’appliquer son expertise dans divers secteurs, notamment l’énergie, les transports, la santé, la production et la gestion de la chaîne logistique.
Titulaire d'un doctorat en ingénierie des systèmes (2000), Andrea Lodi a été professeur titulaire de recherche opérationnelle au Département de génie électrique, électronique et informationnel de l'Université de Bologne. Il coordonne des projets de recherche opérationnelle européens à grande échelle et travaille depuis 2006 comme consultant auprès de l'équipe de recherche et développement CPLEX chez IBM. Il a publié plus de 70 articles dans de grandes revues de programmation mathématique et a été éditeur associé au sein de plusieurs d’entre elles.
Le professeur Lodi a reçu le prix Google 2010 du corps professoral et le prix IBM 2011 du corps professoral. Il a en outre été membre du prestigieux programme Herman Goldstine du centre de recherche IBM Thomas J. Watson en 2005-2006.