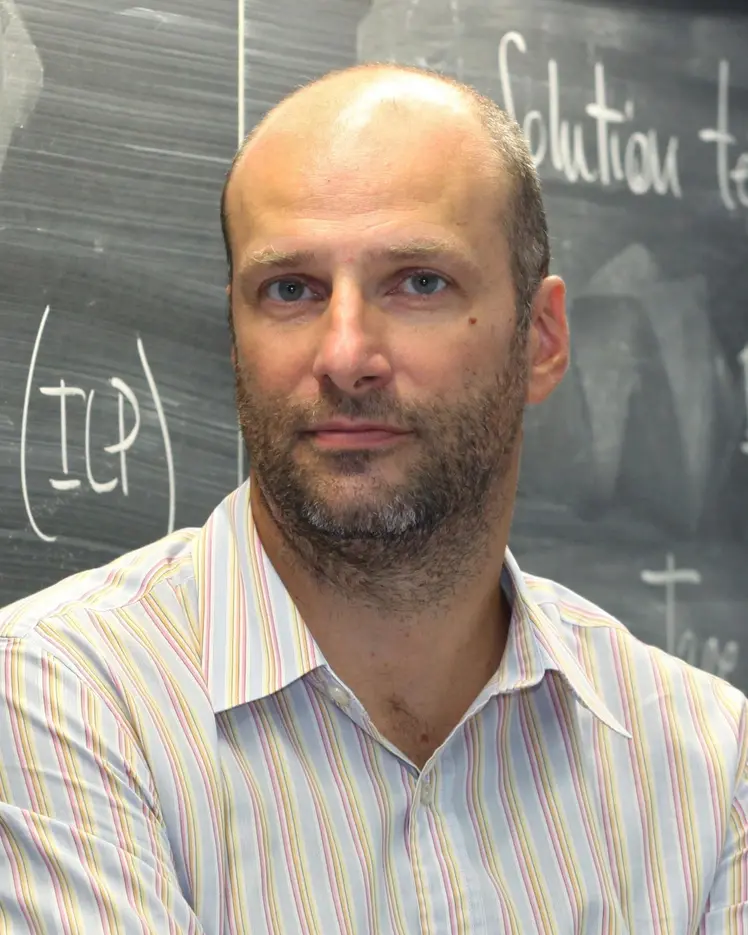
Andrea Lodi
Biography
Andrea Lodi is an adjunct professor in the Department of Mathematics and Industrial Engineering at Polytechnique Montréal, and founder and scientific director of IVADO Labs.
Since 2014, Lodi has held the Canada Excellence Research Chair in Data Science for Real-Time Decision-Making at Polytechnique Montréal. This is Canada’s leading research chair in the field of operations research.
Internationally recognized for his work on mixed linear and nonlinear programming, Lodi is focused on developing new models and algorithms to quickly and efficiently process massive amounts of data from multiple sources. These algorithms and models are expected to lead to the creation of optimized real-time decision-making strategies. The goal of his work as Chair is to apply this expertise in a range of sectors, including energy, transport, health, production and supply chain logistics management.
Lodi holds a PhD in systems engineering (2000) and is a full professor of operations research in the Department of Electrical, Electronic and Information Engineering at the University of Bologna. He coordinates large-scale European operations research projects, and has worked as a consultant for the CPLEX R&D team at IBM since 2006. Lodi has published over seventy articles in major journals in mathematical programming and also served as an associate editor for many of these journals.
His many honours include a 2010 Google Faculty Research Award and a 2011 IBM Faculty Award, and he was a member of the prestigious Herman Goldstine program at the IBM Thomas J. Watson Research Center in 2005–2006.