Nous utilisons des témoins pour analyser le trafic et l’utilisation de notre site web, afin de personnaliser votre expérience. Vous pouvez désactiver ces technologies à tout moment, mais cela peut restreindre certaines fonctionnalités du site. Consultez notre Politique de protection de la vie privée pour en savoir plus.
Paramètre des cookies
Vous pouvez activer et désactiver les types de cookies que vous souhaitez accepter. Cependant certains choix que vous ferez pourraient affecter les services proposés sur nos sites (ex : suggestions, annonces personnalisées, etc.).
-
Cookies essentielsCes cookies sont nécessaires au fonctionnement du site et ne peuvent être désactivés. (Toujours actif)
-
Cookies analyseAcceptez-vous l'utilisation de cookies pour mesurer l'audience de nos sites ?
-
Multimedia PlayerAcceptez-vous l'utilisation de cookies pour afficher et vous permettre de regarder les contenus vidéo hébergés par nos partenaires (YouTube, etc.) ?
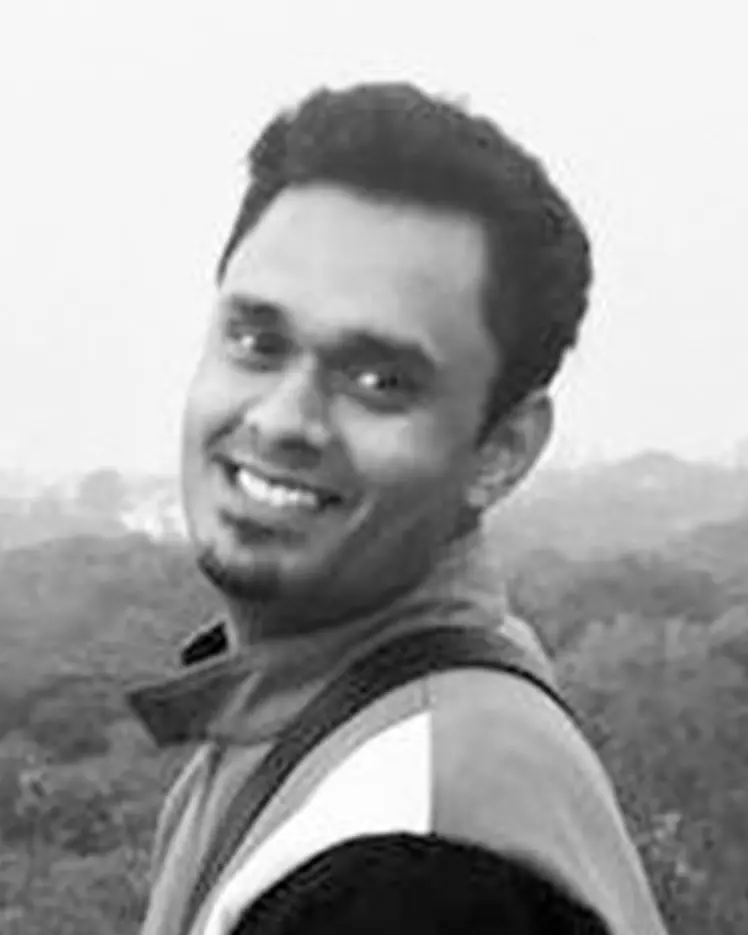