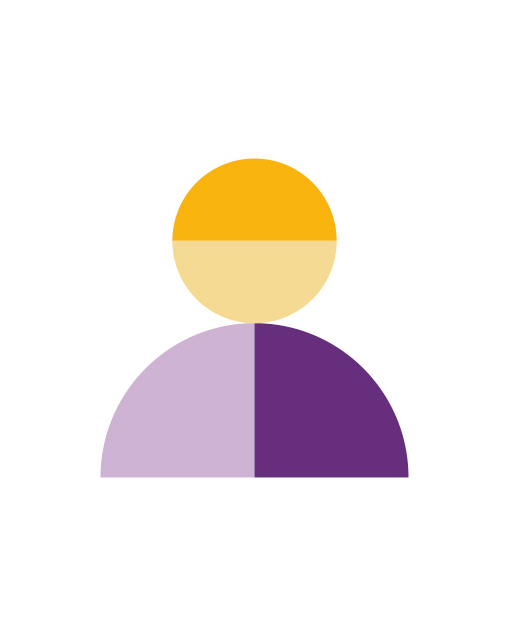
Xue (Steve) Liu
Biography
Xue (Steve) Liu is an associate academic member of Mila – Quebec Artificial Intelligence Institute and full professor at McGill University’s School of Computer Science.
He is also a William Dawson Scholar at McGill, as well as a professor (courtesy appointment) in the Department of Mathematics and Statistics, associate member of the Centre for Intelligent Machines (CIM), and associate member of the Centre for Advanced Systems and Technologies in Communications (SYTACom).
Liu is VP of R&D, chief scientist and co-director of Samsung AI Center Montréal. Before that, he was chief scientist in charge of research and innovation at Tinder Inc., the world’s largest dating and social discovery app, then valued at over US$10 billion.
He is a Fellow of the IEEE and the Canadian Academy of Engineering in addition to being the recipient of many awards, including the 2017 Mitacs Award for Exceptional Leadership – Professor; Outstanding Young Canadian Computer Science Researcher Prize from the Canadian Association of Computer Science (2014); and McGill’s Tomlinson Scientist Award for “recognition of excellence and scientific leadership.” He founded McGill’s Cyber-Physical Intelligence Lab in 2007 and still serves as its director.
Liu also briefly served as Samuel R. Thompson Chair Associate Professor in the Department of Computer Science and Engineering at the University of Nebraska-Lincoln, and worked at Hewlett-Packard Labs in Palo Alto (California) and IBM’s Thomas J. Watson Research Center (New York)