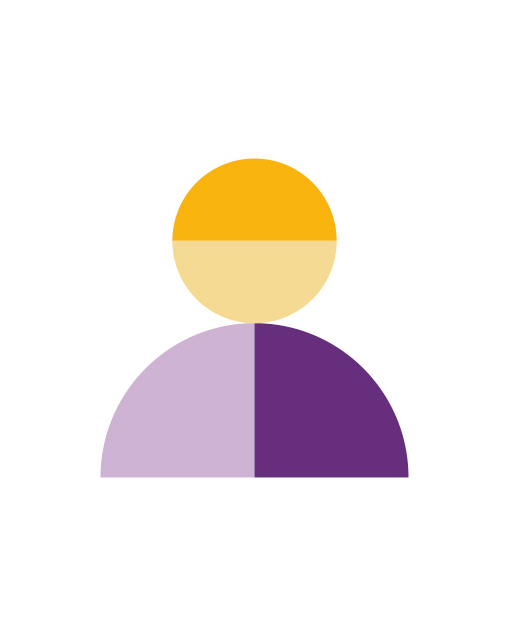
Fernando Diaz
Biography
Fernando Diaz is an associate professor at Carnegie Mellon University's School of Computer Science, a research scientist at Google Pittsburgh, and an adjunct professor in McGill University’s School of Computer Science.
Diaz’s expertise lies in the formal study of the search for small fragments of information in large data sets. His interests include distributed approaches to web-based documentary research, interactive and faceted research, the exploration of temporal models from news and queries, multilingual information research, and graph-based methods.
Diaz’s primary research interest is information retrieval, i.e., the formal study of searching large collections of data for small bits of information. The most familiar form of information retrieval is the web search, where users search a collection of webpages for one or a few relevant webpages. However, information retrieval goes far beyond web searches to include processes like cross-lingual retrieval, personalization, desktop search and interactive retrieval.
Diaz’s research experience includes distributed information retrieval approaches to web searching, interactive and faceted retrieval, mining of temporal patterns from news and query logs, cross-lingual information retrieval, graph-based retrieval methods, and exploiting information from multiple corpora.
For his PhD research, Diaz studied the relationship between document clustering and document scoring for retrieval using methods from machine learning and statistics. As a result, he developed an algorithm for system self-assessment and self-tuning that significantly improves the performance of retrieval algorithms across a variety of corpora.