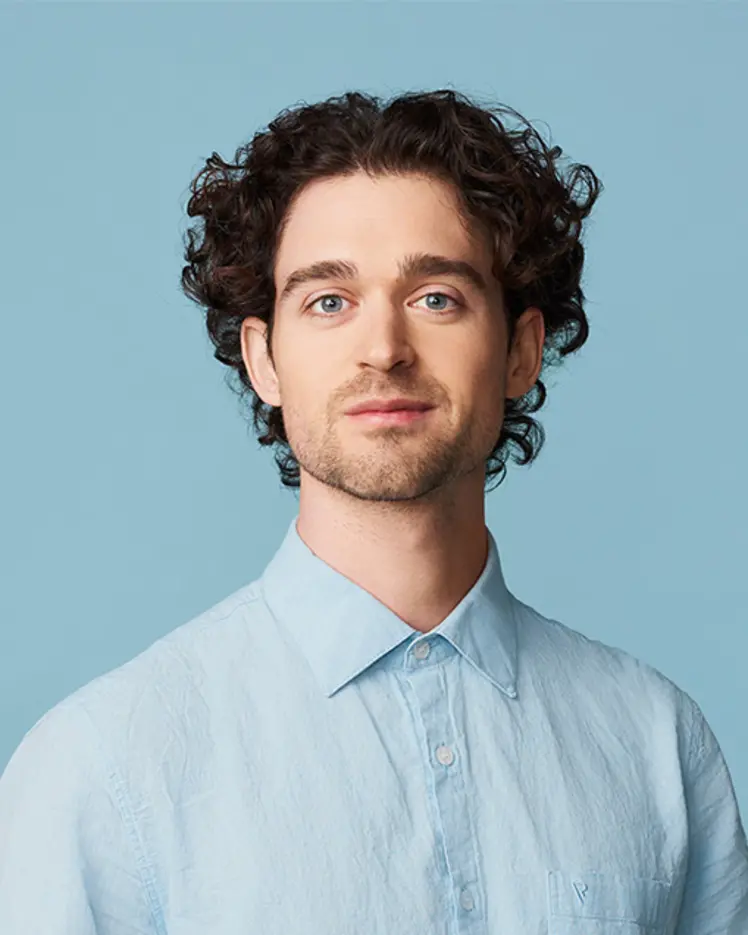
Gauthier Gidel
Biography
I am an assistant professor in the Department of Computer Science and Operations Research (DIRO) at Université de Montréal, a core academic member of Mila – Quebec Artificial Intelligence Institute, and a Canada CIFAR AI Chair.
Previously, I was awarded a Borealis AI Graduate Fellowship, worked at DeepMind and Element AI, and was a Long-Term Visitor at the Simons Institute at UC Berkeley.
My research interests lie at the intersection of game theory, optimization and machine learning.