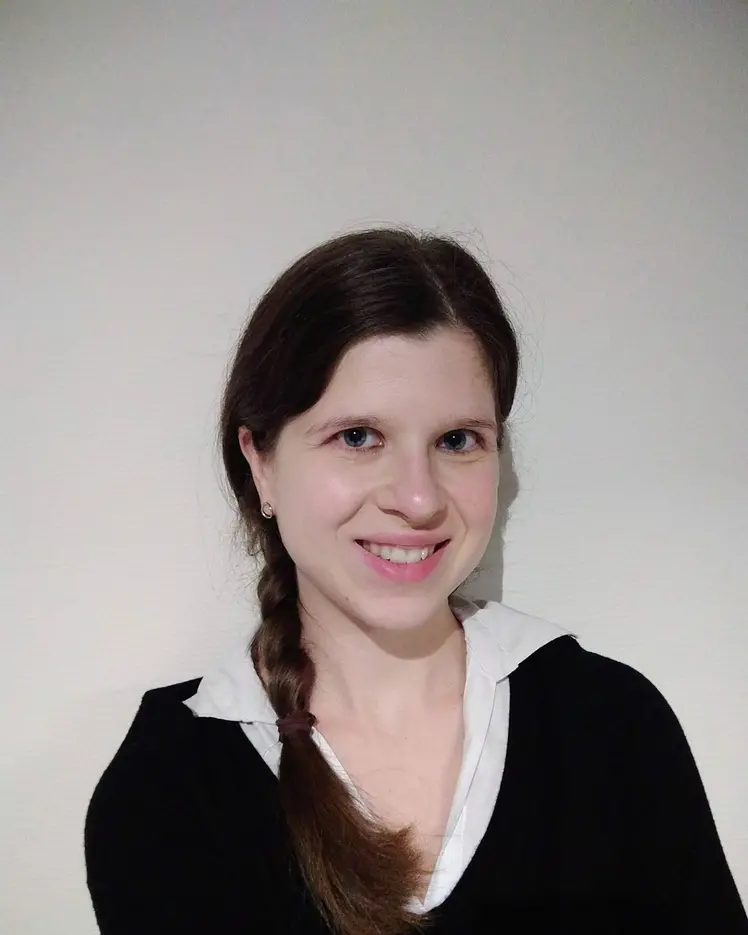
Ayla Rigouts Terryn
Biographie
Ayla Rigouts Terryn est professeure adjointe en technologies de la traduction et en IA au département de linguistique et de traduction de l'Université de Montréal et membre académique associée à Mila. Elle est également chercheuse dans le domaine du traitement du langage naturel (NLP).
Elle a obtenu une maîtrise en traduction à l'université d'Anvers, un doctorat sur l'extraction automatique de terminologie à l'université de Gand et s'est spécialisée dans les technologies linguistiques multilingues en tant que chercheuse principale à la KU Leuven. En tant que linguiste dans le domaine de l'intelligence artificielle, elle se passionne pour l'amélioration de notre compréhension des modèles de langage grâce à des connaissances linguistiques. Elle se concentre principalement sur l'amélioration des technologies dans des contextes multilingues et/ou spécifiques à un domaine.