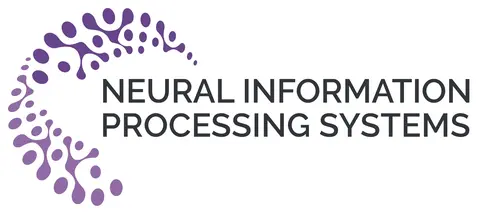
Earlier this October, the Neural Information Processing Systems Conference (NeurIPS) unveiled the complete list of accepted publications for its 34th edition, which will be entirely virtual.
As one of the largest and most important machine learning conferences in the world, the research culture around the submission process has become increasingly competitive. To give an example, the NeurIPS 2020 Chairs have announced that of the 12,115 abstracts submitted, a total of 1,903 have been accepted for this year's conference.
In light of the increasing pressure our researchers are facing, it is important to commemorate all the work our students and professors have done in producing their research and organizing workshops for this conference. Accepted papers from our community cover a range of topics including, but not limited to, novel continual model-agnostic meta-learning approaches, solutions to oversmoothness in graph convolutional networks, and two policy regularization methods in multi-agent deep reinforcement learning.
Below is the complete list of publications, as well as an overview of the workshops co-organized by Mila members:
- Untangling tradeoffs between recurrence and self-attention in neural networks
- Top-k Training of GANs: Improving GAN Performance by Throwing Away Bad Samples
- Hybrid Models for Learning to Branch
- The LoCA Regret: A Consistent Metric to Evaluate Model-Based Behavior in Reinforcement Learning
- Your GAN is Secretly an Energy-based Model and You Should use Discriminator Latent Sampling
- Counterexample-Guided Learning of Monotonic Neural Networks
- Adversarial Example Games
- Online Fast Adaptation and Knowledge Accumulation: A New Approach to Continual Learning
- Learning Dynamic Belief Graphs to Generalize on Text-Based Games
- Measuring Systematic Generalization in Neural Proof Generation with Transformers
- Black-Box Certification with Randomized Smoothing: A Functional Optimization Based Framework
- Forethought and Hindsight in Credit Assignment
- Real World Games Look like Spinning Tops
- Neumann Networks: Differential programming for supervised learning with missing values
- Training Linear Finite-State Machines
- RL Unplugged: Benchmarks for Offline Reinforcement Learning
- Graph Policy Network for Transferable Active Learning on Graphs
- Towards Interpretable Natural Language Understanding with Explanations as Latent Variables
- Promoting Coordination through Policy Regularization in Multi-Agent Deep Reinforcement Learning
- Scattering GCN: Overcoming Oversmoothness in Graph Convolutional Networks
- In search of robust measures of generalization
- An Equivalence between Loss Functions and Non-Uniform Sampling in Experience Replay
- Learning Graph Structure with A Finite-State Automaton Layer
- Unsupervised Learning of Dense Visual Representations
- Equivariant Networks for Hierarchical Structures
- Symbols: Probing Learning Algorithms with Synthetic Datasets
- Explicit Regularization is Stronger than Implicit Bias: A Study of SGD around Bad Global Minima
- Novelty Search in representational space for sample efficient exploration
- La-MAML: Look-ahead Meta Learning for Continual Learning
- Adversarial Soft Advantage Fitting: Imitation Learning Without Policy Optimization
- Differentiable Causal Discovery from Interventional Data
- Reward Propagation using Graph Convolutional Networks
- Uncovering the Topology of Time-Varying fMRI Data using Cubical Persistence
Giancarlo Kerg, Bhargav Kanuparthi, Anirudh Goyal, Kyle Goyette, Yoshua Bengio, Guillaume Lajoie
https://arxiv.org/abs/2006.09471
Samarth Sinha, Zhengli Zhao, Anirudh Goyal, Colin Raffel, Augustus Odena
https://arxiv.org/abs/2002.06224
Prateek Gupta, Maxime Gasse, Elias B. Khalil, M. Pawan Kumar, Andrea Lodi, Yoshua Bengio
https://arxiv.org/abs/2006.15212
Harm van Seijen, Hadi Nekoei, Evan Racah, Sarath Chandar
https://arxiv.org/abs/2007.03158
Tong Che, Ruixiang Zhang, Jascha Sohl-Dickstein, Hugo Larochelle, Liam Paull, Yuan Cao, Yoshua Bengio
https://arxiv.org/abs/2003.06060
Aishwarya Sivaraman, Golnoosh Farnadi, Todd Millstein, Guy Van den Broeck
https://arxiv.org/abs/2006.08852
Joey Bose, Gauthier Gidel, Hugo Berard, Andre Cianflone, Pascal Vincent, Simon Lacoste-Julien, William Hamilton
https://arxiv.org/abs/2007.00720
Massimo Caccia, Pau Rodriguez, Oleksiy Ostapenko, Fabrice Normandin, Min Lin, Lucas Caccia, Issam Laradji, Irina Rish, Alexandre Lacoste, David Vazquez, Laurent Charlin
https://arxiv.org/abs/2003.05856
Ashutosh Adhikari, Xingdi Yuan, Marc-Alexandre Côté, Mikulás Zelinka, Marc-Antoine Rondeau, Romain Laroche, Pascal Poupart, Jian Tang, Adam Trischler, William Hamilton
https://arxiv.org/abs/2002.09127
Nicolas Gontier, Koustuv Sinha, Siva Reddy, Christopher Pal
https://arxiv.org/abs/2009.14786
Dinghuai Zhang, Mao Ye, Chengyue Gong, Zhanxing Zhu, Qiang Liu
https://arxiv.org/abs/2002.09169
Veronica Chelu, Doina Precup, Hado van Hasselt
Wojciech Marian Czarnecki, Gauthier Gidel, Brendan Tracy, Karl Tuyls, Shayegan Omidshafiefi, David Balduzzi, Max Jaderberg
https://arxiv.org/abs/2004.09468
Marine Le Morvan, Julie Josse, Thomas Moreau, Erwan Scornet, Gaël Varoquaux
https://arxiv.org/abs/2007.01627
Arash Ardakani, Amir Ardakani, Warren Gross
Caglar Gulcehre, Ziyu Wang, Alexander Novikov, Tom Le Paine, Sergio Gomez Colmenarejo, Konrad Zolna, Rishabh Agarwal, Josh Merel, Daniel Mankowitz, Cosmin Paduraru, Gabriel Dulac-Arnold, Jerry Li, Mohammad Norouzi, Matt Hoffman, Ofir Nachum, George Tucker, Nicolas Heess, Nando de Freitas
https://arxiv.org/abs/2006.13888
Shengding Hu, Zheng Xiong, Xingdi Yuan, Marc-Alexandre Côté, Zhiyuan Liu, Jian Tang
https://arxiv.org/abs/2006.13463
Wangchunshu Zhou, Jinyi Hu, Hanlin Zhang, Xiaodan Liang, Maosong Sun, Chenyan Xiong, Jian Tang
Julien Roy, Paul Barde, Félix Harvey, Derek Nowrouzezahrai, Christopher Pal
https://arxiv.org/abs/1908.02269
Yimeng Min, Frederik Wenkel, Guy Wolf
https://arxiv.org/abs/2003.08414
Gintare Karolina Dziugaite, Alexandre Drouin, Brady Neal, Nitarshan Rajkumar, Ethan Caballero, Linbo Wang, Ioannis Mitliagkas, Daniel Roy
Scott Fujimoto, David Meger, Doina Precup
Daniel D. Johnson, Hugo Larochelle, Daniel Tarlow
https://arxiv.org/abs/2007.04929
Pedro O. Pinheiro, Amjad Almahairi, Ryan Benmalek, Florian Golemo, Aaron Courville
Renhao Wang, Marjan Albooyeh, Siamak Ravanbakhsh
Alexandre Lacoste, Pau Rodríguez, Frederic Branchaud-Charron, Massimo Caccia, Issam Hadj Laradji, Alexandre Drouin, Matthew Craddock, Laurent Charlin, David Vázquez
https://arxiv.org/abs/2009.06415
Shengchao Liu, Dimitris Papailiopoulos, Dimitris Achlioptas
Ruo Yu Tao, Vincent François-Lavet, Joelle Pineau
https://arxiv.org/abs/2009.13579
Gunshi Gupta, Karmesh Yadav, Liam Paull
https://arxiv.org/abs/2007.13904
Paul Barde, Julien Roy, Wonseok Jeon, Joelle Pineau, Christopher Pal, Derek Nowrouzezahrai
https://arxiv.org/abs/2006.13258
Philippe Brouillard, Sébastien Lachapelle, Alexandre Lacoste, Simon Lacoste-Julien, Alexandre Drouin
https://arxiv.org/abs/2007.01754
Martin Klissarov and Doina Precup
https://arxiv.org/abs/2010.02474
Bastian Rieck, Tristan Yates, Christian Bock, Karsten Borgwardt, Guy Wolf, Nicholas Turk-Browne, Smita Krishnaswamy
Workshops:
- Tackling Climate Change with Machine Learning
- Topological Data Analysis and Beyond
- Differential Geometry meets Deep Learning (DiffGeo4DL)
- Differentiable vision, graphics, and physics applied to machine learning
- Resistance AI
- Biological and Artificial Reinforcement Learning
- ML Retrospectives, Surveys & Meta-Analyses
- Object Representations for Learning and Reasoning
- Algorithmic Fairness through the Lens of Causality and Interpretability
- AI for Earth Sciences
- ML4H: Machine Learning for Health
- Women in Machine Learning
- Offline Reinforcement Learning
Tegan Maharaj, Priya Donti, Lynn Kaack, Alexandre Lacoste, Andrew Ng, John Platt, Jennifer Chayes, Yoshua Bengio
https://www.climatechange.ai/events/neurips2019.html
Bastian Rieck, Frederic Chazal, Smita Krishnaswamy, Roland Kwitt, Karthikeyan Natesan Ramamurthy, Yuhei Umeda, and Guy Wolf
https://sites.google.com/view/diffgeo4dl/
Krishna Murthy, Kelsey Allen, Victoria Dean, Johanna Hansen, Shuran Song, Florian Shkurti, Liam Paull, Derek Nowrouzezahrai, Josh Tenenbaum
https://montrealrobotics.ca/diffcvgp/
Mattie Tesfaldet, J Khadijah Abdurahman, William Agnew, Abeba Birhane, Elliot Creager, Agata Foryciarz, Pratyusha Ria Kalluri, Sayash Kapoor, Raphael Gontijo Lopes, Manuel Sabin, Marie-Therese Png, Maria Skoularidou, Ramon Vilarino, Rose E. Wang
https://sites.google.com/view/resistance-ai-neurips-20/home
Raymond Chua, Feryal Behbahani, Sara Zannone, Doina Precup, Ida Momennejad, Blake Richards, Rui Ponte Costa
https://sites.google.com/view/biologicalandartificialrl
Chhavi Yadav, Prabhu Pradhan, Abhishek Gupta, Peter Henderson, Ryan Lowe, Jessica Forde, Jesse Dodge, Mayoore Jaiswal, Joelle Pineau
https://ml-retrospectives.github.io/neurips2020/
William Agnew, Rim Assouel, Michael Chang, Antonia Creswell, Eliza Kosoy, Aravind Rajeswaran, Sjoerd van Steenkiste
https://orlrworkshop.github.io/index.html
Golnoosh Farnadi, Awa Dieng, Jessica Schrouff, Matt Kusner, Fernando Diaz
Natasha Dudek, S. Karthik Mukkavilli, Johanna Hansen, Kelly Kochanski, S. Karthik Mukkavilli, Tom Beucler, Karthik Kashinath, Mayur Mudigonda, Amy McGovern
https://ai4earthscience.github.io/neurips-2020-workshop/organizers.html
Benjamin Akera, Emily Alsentzer, Oliver J. Bear, Ioana Bica, Irene Chen, Fabian Falck, Stephanie Hyland, Dani Kyasseh, Matthew McDermott, Ehi Nosakhare, Charles Onu, Stephen Pfohl, Aahlad Manas Puli, Suproteem Sarkar, Allen Schmaltz
https://ml4health.github.io/2020/pages/organizers.html
Amy Zhang, Elizabeth Wood, Mel Woghiren, Kristy Choi, Raquel Aoki, Judy Hanwen Shen, Belén Saldías, Xenia Miscouridou, Krystal Maughan, Xinyi Chen, Erin Grant
https://wimlworkshop.org/neurips2020/contact-us/
Rishabh Agarwal, Aviral Kumar, George Tucker, Doina Precup, Lihong Li