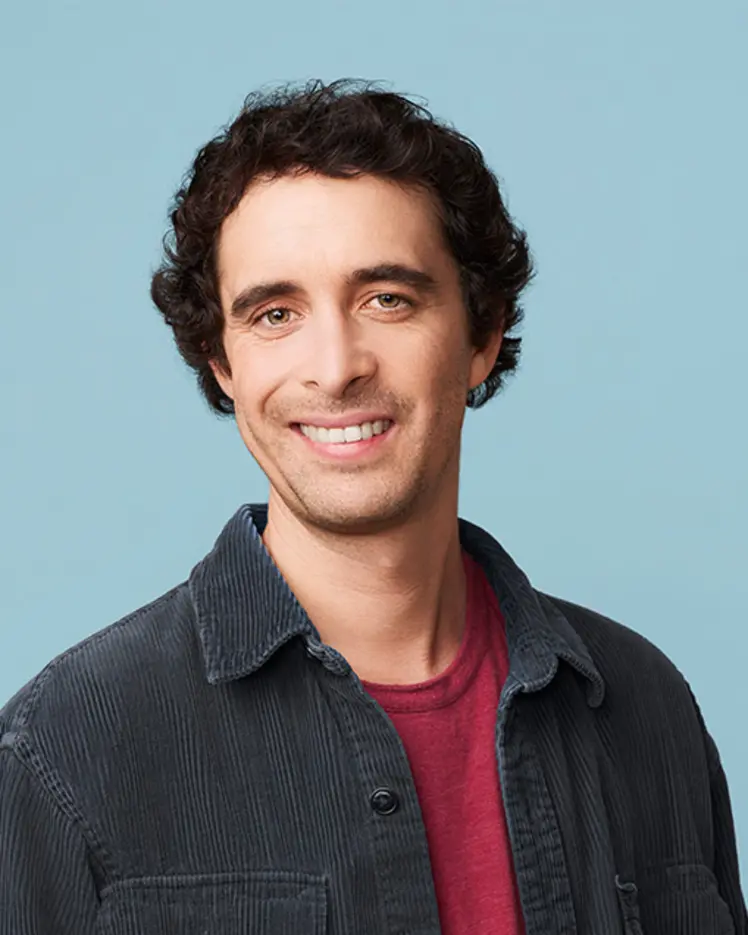
Pierre-Luc Bacon
Biography
Pierre-Luc Bacon is an assistant professor at Université de Montréal in the Department of Computer Science and Operations Research (DIRO). He is also a core academic member of Mila – Quebec Artificial Intelligence Institute and IVADO, and holds a Facebook CIFAR AI Chair. Bacon leads a research group that investigates the challenges posed by the curse of the horizon in reinforcement learning and optimal control.