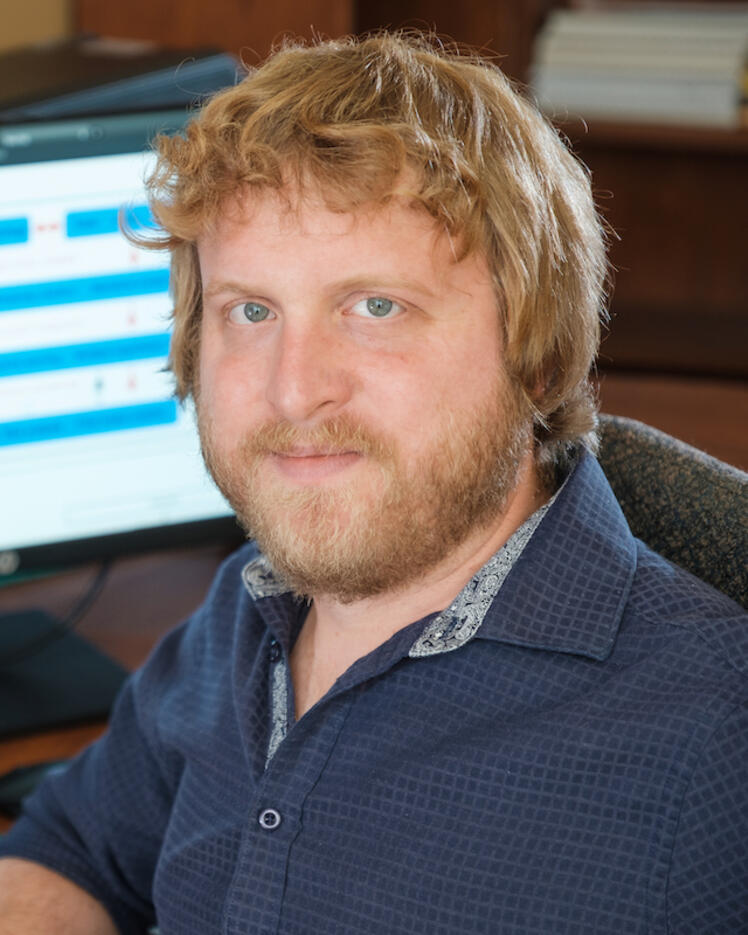
Martin Vallières
Biographie
Martin Vallières est professeur adjoint au Département d'informatique de l'Université de Sherbrooke et titulaire d'une chaire en IA Canada-CIFAR depuis avril 2020. Il a obtenu un doctorat en physique médicale de l'Université McGill en 2017, et a suivi une formation postdoctorale en France et aux États-Unis en 2018 et 2019. Il est expert dans le domaine de la radiomique et de l'apprentissage automatique en oncologie. Au cours de sa carrière, il a développé de multiples modèles de prédiction pour différents types de cancer. Son principal intérêt de recherche consiste désormais en la modélisation à base de graphes de données médicales hétérogènes pour améliorer la médecine de précision.