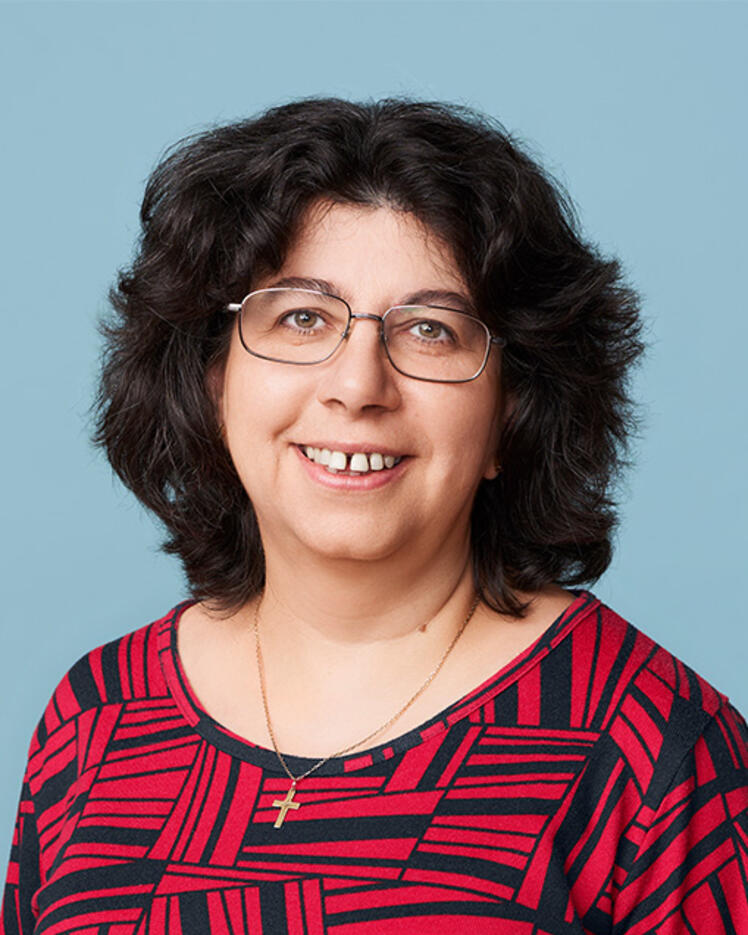
Doina Precup
Biography
Doina Precup combines teaching at McGill University with fundamental research on reinforcement learning, in particular AI applications in areas of significant social impact, such as health care. She is interested in machine decision-making in situations where uncertainty is high.
In addition to heading the Montreal office of Google DeepMind, Precup is a Senior Fellow of the Canadian Institute for Advanced Research and a Fellow of the Association for the Advancement of Artificial Intelligence.
Her areas of speciality are artificial intelligence, machine learning, reinforcement learning, reasoning and planning under uncertainty, and applications.