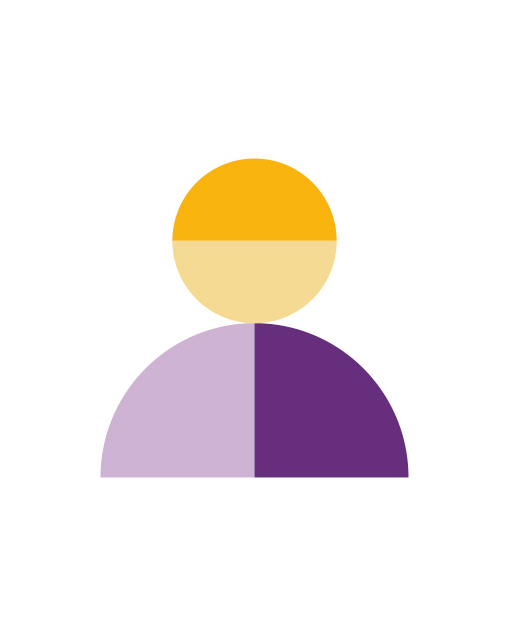
Adriana Romero Soriano
Biography
Adriana Romero-Soriano is a research scientist in the Fundamental AI Research (FAIR) team at Meta, adjunct professor at McGill University, core industry member of Mila – Quebec Artificial Intelligence Institute and a Canada CIFAR AI Chair.
Romero-Soriano’s research lies at the intersection of generative models, computer vision and responsible AI, while her most recent work focuses on improving the quality, controllability, consistency and representation diversity of visual content creation systems.
She received her PhD from the University of Barcelona, where she worked with Carlo Gatta, and then spent two years as a postdoctoral researcher at Mila with Yoshua Bengio.