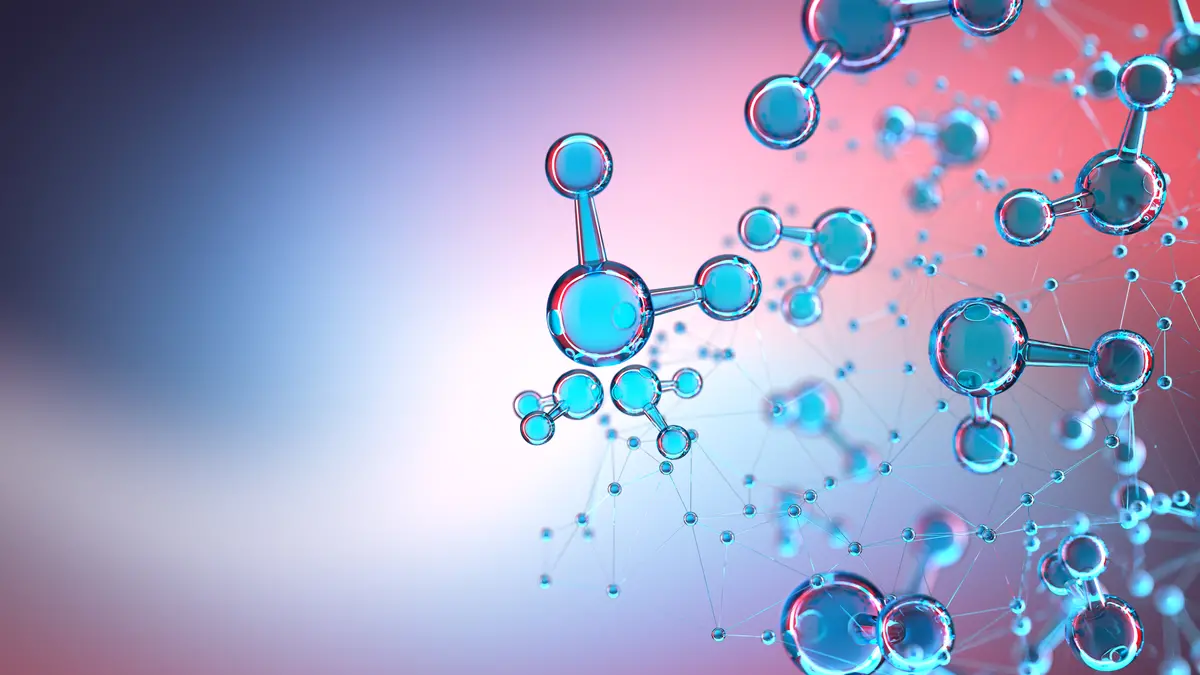
Introducing a powerful open-source protein language model
AI can help scientists find new therapeutic proteins and develop new drugs thanks to language models similar to the ones used to generate text. However, current state-of-the-art protein language models are expensive to develop and complex to deploy for academic researchers. Our newest protein language model, AMPLIFY (Amgen-Mila Protein Language model for InFerence and discoverY), aims to change that by offering a smaller, more efficient open-source model to reduce the barriers to access cutting-edge protein discovery tools.
Proteins are the fundamental building blocks of living organisms. While DNA and RNA serve as the blueprints of life, proteins act as the structural and functional materials. They also play a vital role as drugs — notably as antibodies — in therapies for autoimmune diseases and cancer.
New tools that enhance our understanding of proteins are crucial for advancing research and designing more effective drugs. Recently, AI researchers have made significant progress in understanding and designing complex proteins, notably through the development of protein language models.
A more efficient and accessible way to discover proteins
Much like how large language models can generate valid and intelligible text from a simple prompt, these protein language models can predict and analyze protein structures and functions by interpreting amino acid sequences.
In the context of drug discovery and medical research, this advancement has the potential to dramatically reduce the time and costs associated with finding promising drug candidates for wet lab experiments.
If a lab can discriminate early on between proteins that are suitable or unsuitable for real-world experiments, it can avoid expensive mistakes, as the cost of abandoning a drug candidate grows as it progresses through the development pipeline. This allows efforts and resources to be concentrated on the most promising candidates, increasing the likelihood of success.
Current protein language models are expensive to use and do not provide a convenient or cost-effective way for most research labs to conduct experiments with their own training datasets.
We started with a simple but ambitious question: could we provide smaller players with greater access to protein language models and level the playing field for researchers around the world?
Less is more
Major players already exist in this field, notably AlphaFold, created by Google DeepMind, and the ESM protein language models created by Meta. ESM-2 currently stands as the sequence-only best protein language model, but with 15 billion parameters, we estimate that training the largest ESM-2 costs over a million dollars, far out of reach for most labs to reproduce.
Yet ESM-2 and other existing protein language models process data in a way that is largely biased towards outliers and non-proteins, requiring immense amounts of power and countless parameters to deliver useful results. This raises questions about their efficiency, and about whether their enormous size, prohibitive cost, and gate-kept data pipelines are necessary.
In collaboration with Amgen, we addressed those dataset biases and implemented techniques from the latest language models to create AMPLIFY, our own cutting-edge protein language model. We found that AMPLIFY not only competes with, but even surpasses ESM2 on some tasks, despite having 43 times fewer parameters, at only 350 million. It also requires 17 times less compute to train, and is up to 2,000 times faster at predicting.
AMPLIFY raises an exciting question: is scaling necessary to create better protein generative models, or is this an opportunity to prioritize quality over quantity?
A true open-science approach
Innovation doesn’t always mean building bigger models — it’s also about building more efficient ones. Through this project, we were able to achieve similar — and sometimes even better — results in protein prediction tasks, without the prohibitive costs and computational burdens associated with existing methods.
AMPLIFY will therefore allow labs around the world to engage in cutting-edge protein research and speed up the process of designing new therapeutic drugs.
Ultimately, we made a point of adopting a true open-science approach. Committed to democratizing science, we are releasing AMPLIFY’s pre-training codebase, data, and model checkpoints under an open-source license for the scientific community.
Until now, much of the machine learning community has struggled to develop new models because it requires a lot of data, resources, and above all, money. Thanks to this close collaboration between AI and biological experts, we hope that more research groups can now use this compact and efficient state-of-the-art model to develop their own protein sequence models and push the boundaries of scientific discovery.
AMPLIFY is the result of the collaboration between Mila, Amgen, and the Chandar Research Lab.