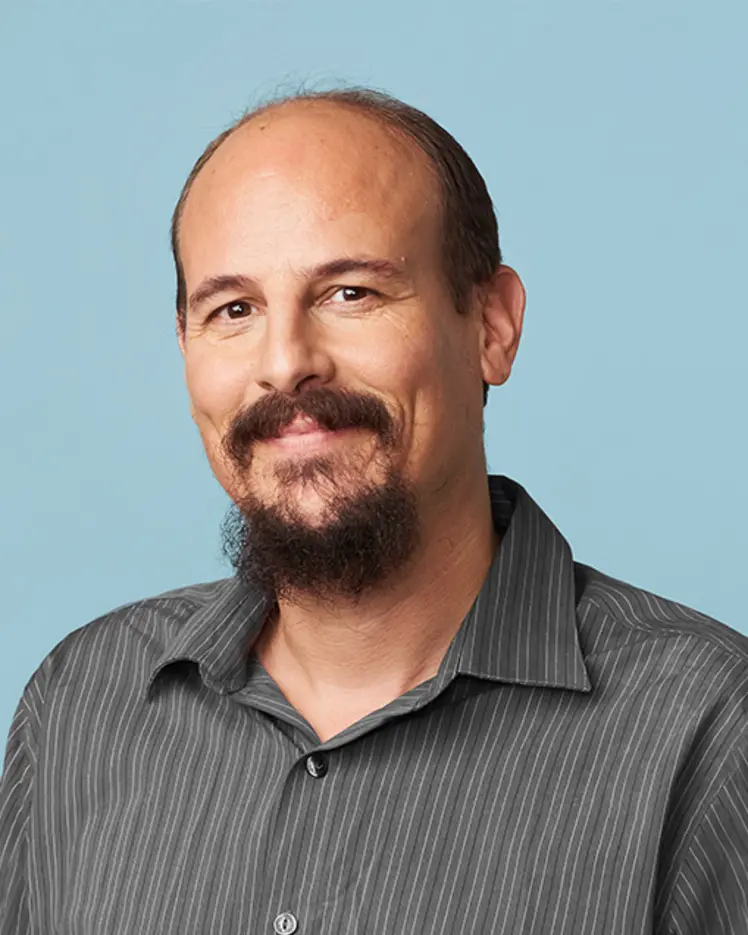
Guillaume Rabusseau
Biography
I have been an assistant professor at Mila – Quebec Artificial Intelligence Institute and in the Department of Computer Science and Operations Research (DIRO) at Université de Montréal (UdeM) since September 2018. I was awarded a Canada CIFAR AI Chair in March 2019. Before joining UdeM, I was a postdoctoral research fellow in the Reasoning and Learning Lab at McGill University, where I worked with Prakash Panangaden, Joelle Pineau and Doina Precup.
I obtained my PhD in 2016 from Aix-Marseille University (AMU) in France, where I worked in the Qarma team (Machine Learning and Multimedia) under the supervision of François Denis and Hachem Kadri. I also obtained my MSc in fundamental computer science and my BSc in computer science from AMU. I am interested in tensor methods for machine learning and in designing learning algorithms for structured data by leveraging linear and multilinear algebra (e.g., spectral methods).