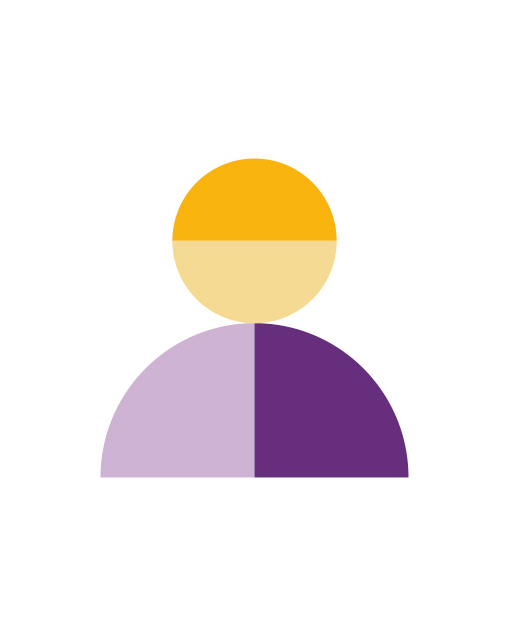
Eugene Belilovsky
Biography
Eugene Belilovsky is an assistant professor in the Department of Computer Science and Software Engineering at Concordia University.
He is also an associate academic member of Mila – Quebec Artificial Intelligence Institute and an adjunct professor at Université de Montréal.
Belilovsky’s research specialties lie in computer vision and deep learning. His current interests include continual learning and few-shot learning, along with applications of these aspects at the intersection of computer vision and language processing.