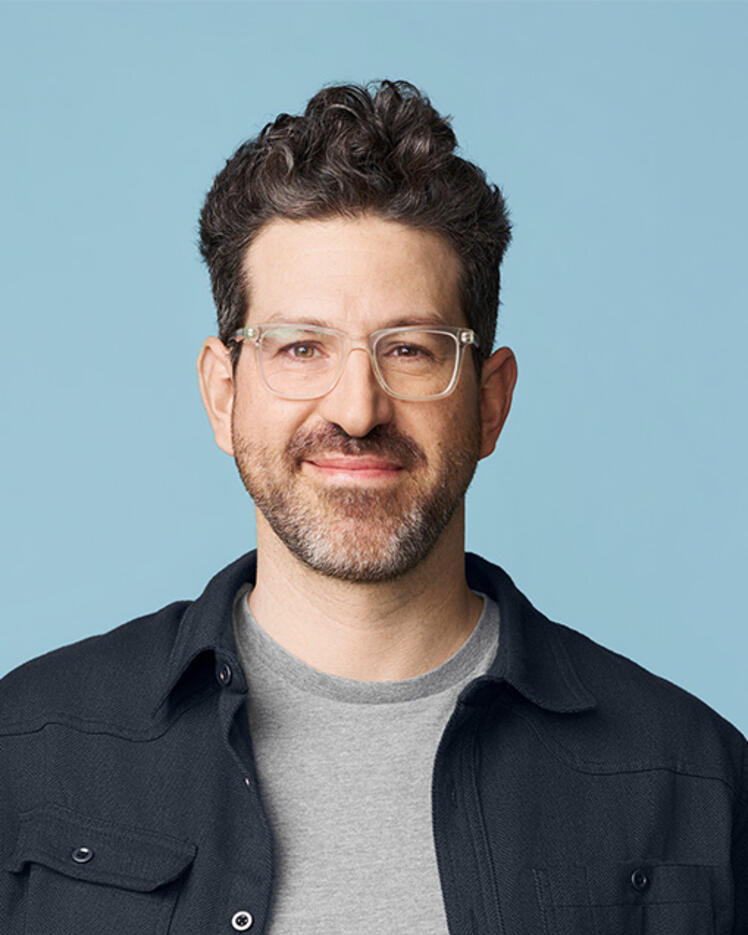
Guillaume Lajoie
Biographie
Guillaume Lajoie est professeur adjoint au Département de mathématiques et de statistique (DMS) de l'Université de Montréal et membre académique principal de Mila – Institut québécois d’intelligence artificielle. Il est également chercheur boursier du Fonds de recherche du Québec - Santé (FRQS). Auparavant, il a été chercheur postdoctoral à l'Institut de dynamique et d'auto-organisation Max-Planck et à l'Institut de neuro-ingénierie de l'Université de Washington. Il a obtenu son doctorat à l'Université de Washington (Seattle), au Département de mathématiques appliquées.
Ses recherches, à l’intersection de l’IA et des neurosciences, se penchent sur des questions liées aux dynamiques et aux calculs des réseaux neuronaux, avec certaines applications à la neuro-ingénierie. Ses travaux récents comprennent le développement de biais inductifs pour une meilleure propagation de l’information dans les réseaux récurrents, ainsi que le développement d'algorithmes permettant d’optimiser les interfaces cerveau-machine bidirectionnelles.