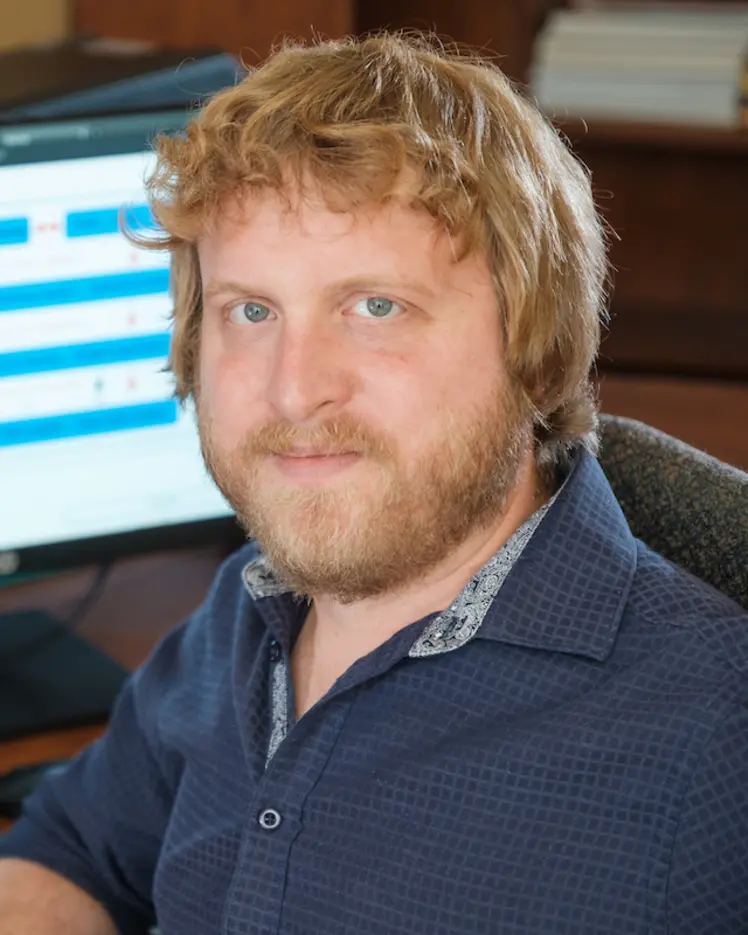
Martin Vallières
Biography
Martin Vallières is an assistant professor in the Department of Computer Science at Université de Sherbrooke and a Canada CIFAR AI Chair since April 2020.
He received a PhD in medical physics from McGill University in 2017, and completed postdoctoral training in France and the U.S. in 2018 and 2019.
Vallières is an expert in the field of radiomics and machine learning in oncology. Over the course of his career, he has developed multiple prediction models for different types of cancers. His main research interest is now focused on the graph-based modelling of heterogeneous medical data for improved precision medicine.