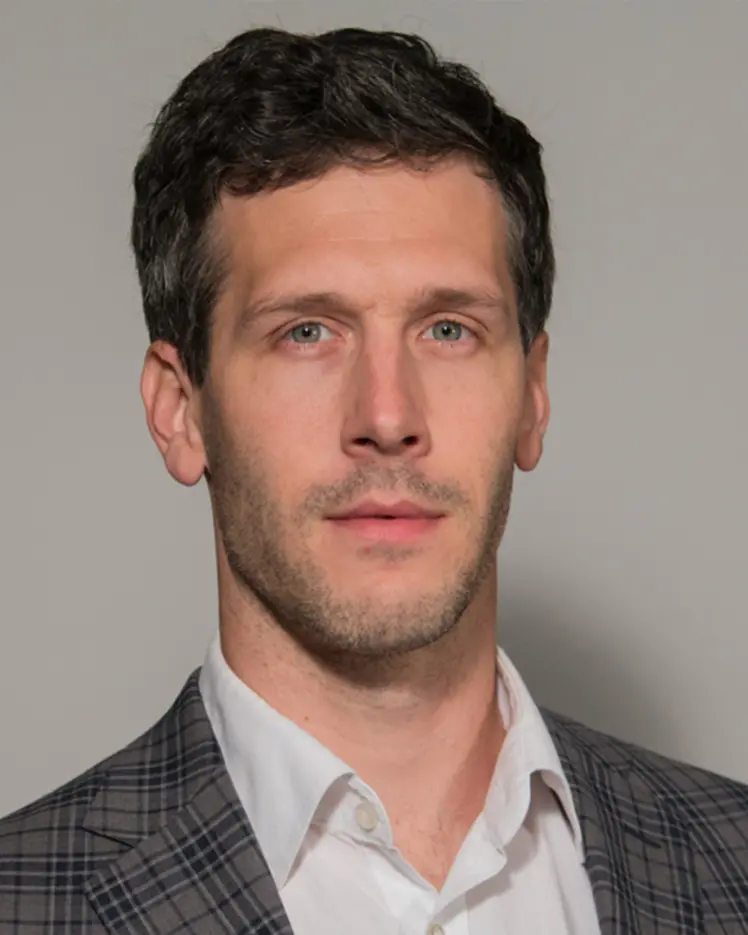
Liam Paull
Biography
Liam Paull is an associate professor at Université de Montréal and co-leads the Montréal Robotics and Embodied AI Lab (REAL). His lab focuses on a variety of robotics problems, including building representations of the world for such applications as simultaneous localization and mapping, modelling uncertainty, and building better workflows to teach robotic agents new tasks through, for example, simulation or demonstration.
Previously, Paull was a research scientist in the Computer Science and Artificial Intelligence Laboratory (CSAIL) at the Massachusetts Institute of Technology (MIT), where he led the autonomous car project funded by the Toyota Research Institute (TRI). He completed a postdoc with the Marine Robotics Group at MIT, where he worked on Simultaneous Localization and Mapping (SLAM) for underwater robots.
His PhD from the University of New Brunswick in 2013 focused on robust and adaptive planning for underwater vehicles. He is also the co-founder and director of the Duckietown Foundation, which is dedicated to making engaging robotics learning experiences accessible to everyone.