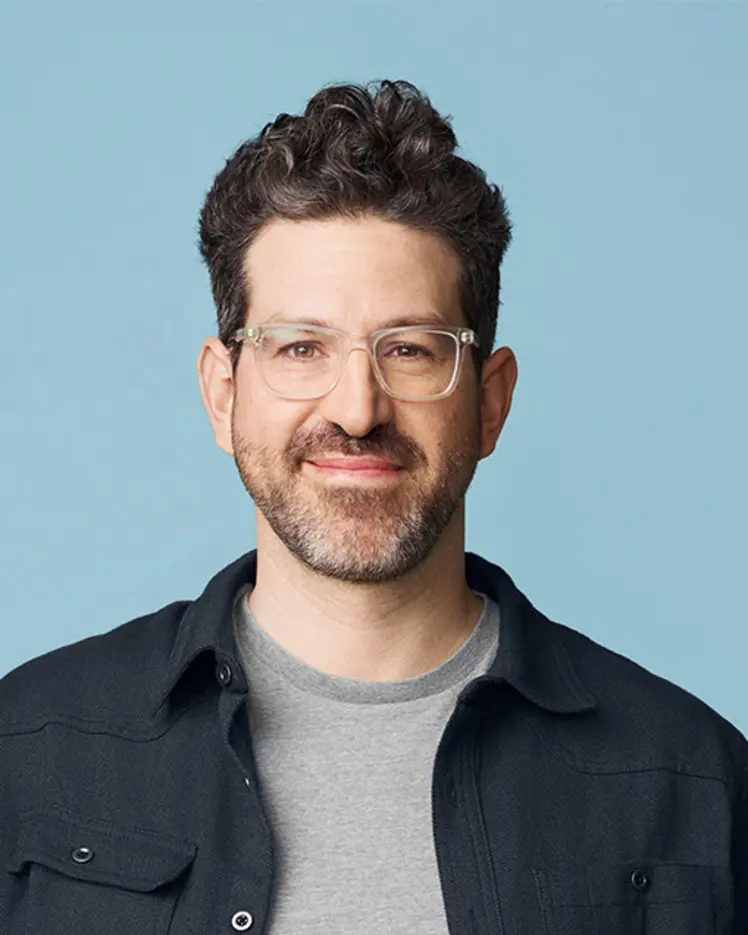
Guillaume Lajoie
Biography
Guillaume Lajoie is an Associate professor in the Department of Mathematics and Statistics at Université de Montréal and a core academic member of Mila – Quebec Artificial Intelligence Institute. He is also a Fonds de recherche du Québec - Health Research Scholar and holds a Tier 2 Canada Research Chair in Neural Computation and Interfacing.
Previously, Lajoie was a postdoctoral fellow at the Max Planck Institute for Dynamics and Self-Organization in Germany and at the University of Washington’s Institute for Neuroengineering. He obtained his PhD from the Department of Applied Mathematics at the University of Washington (Seattle).
Lying at the intersection of AI and neuroscience, Lajoie’s research pursues questions surrounding neural network dynamics and computations, which has potential applications to neuroengineering.
Recent work has focused on the development of architectural inductive biases for information propagation in recurrent networks, as well as the development of algorithms and models for bidirectional brain-machine interface optimization.