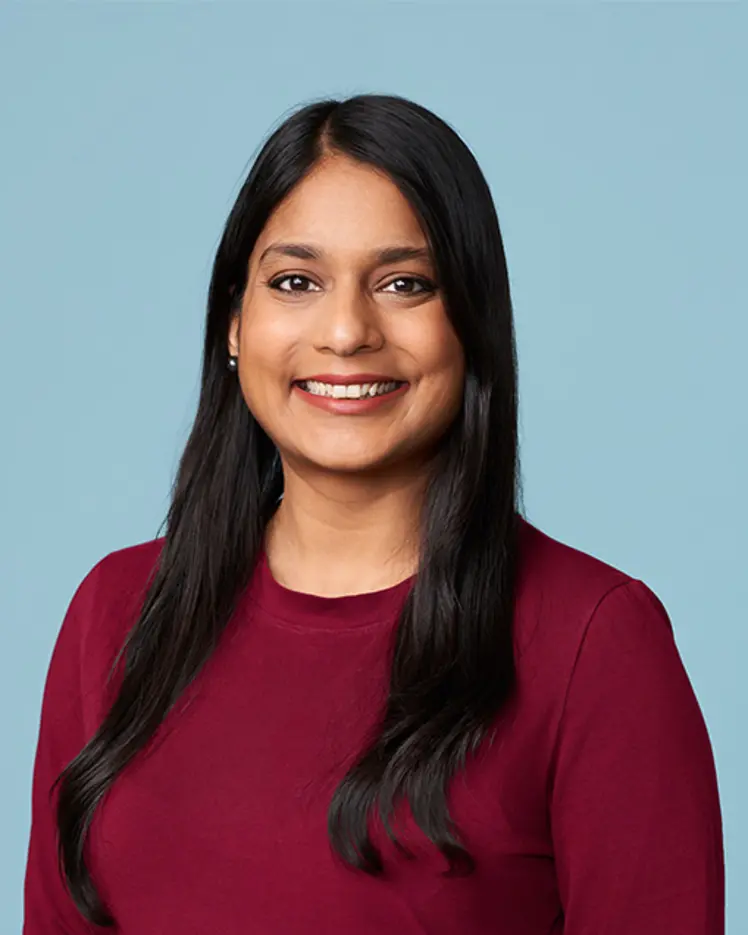
Aishwarya Agrawal
Biography
Aishwarya Agrawal is an assistant professor in the Department of Computer Science and Operations Research at Université de Montréal, a Canada CIFAR AI Chair, and a core academic member of Mila – Quebec Artificial Intelligence Institute.
Agrawal also works as a research scientist one day a week at DeepMind. Previously, she held this position full time (August 2019 to December 2020). She completed her PhD in August 2019 at Georgia Tech, where she worked with Dhruv Batra and Devi Parikh.
Her research interests lie at the intersection of the following sub-disciplines of AI: computer vision, deep learning and natural language processing. The focus is developing AI systems that can ‘see’ (i.e., understand the contents of an image: who, what, where, doing what?) and ‘talk’ (i.e., communicate the understanding to humans in free-form natural language).
Aishwarya has received many awards and scholarships: Georgia Tech 2020 Sigma Xi Best PhD Thesis Award, 2020 Georgia Tech College of Computing Dissertation Award, 2019 Google Fellowship (declined due to graduation), 2019–2020 Facebook Fellowship (declined due to graduation) and 2018–2019 NVIDIA Graduate Fellowship. She was one of two runners-up in the 2019 AAAI/ACM SIGAI Dissertation Award, and was selected as a 2018 Rising Star in EECS.
She holds a bachelor's degree in electrical engineering with a minor in computer science and engineering from the Indian Institute of Technology Gandhinagar (2014).