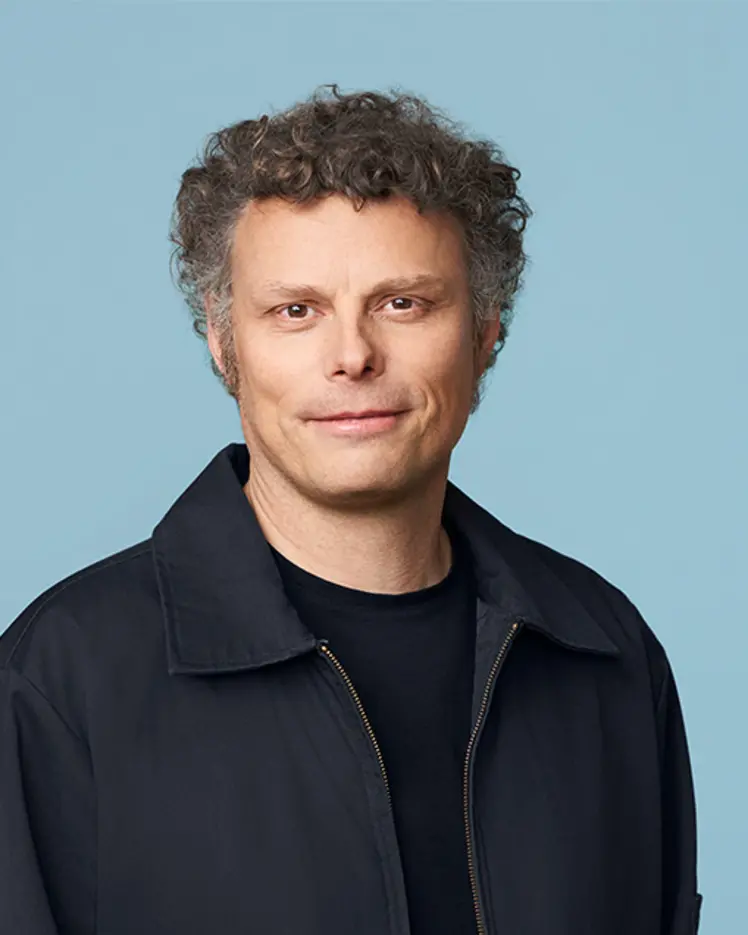
Chris Pal
Biography
Christopher Pal is a Canada CIFAR AI Chair, full professor at Polytechnique Montréal and adjunct professor in the Department of Computer Science and Operations Research (DIRO) at Université de Montréal. He is also a Distinguished Scientist at ServiceNow Research.
Pal has been involved in AI and machine learning research for over twenty-five years and has published extensively on large-scale language modelling methods and generative modelling techniques. He has a PhD in computer science from the University of Waterloo.