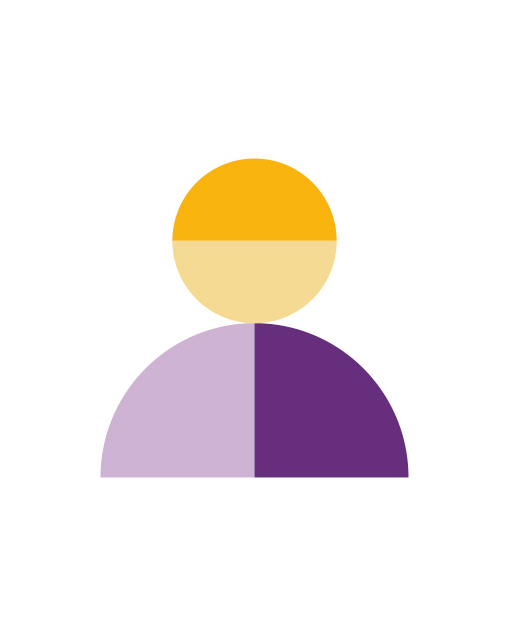
Laurence Perreault-Levasseur
Biography
Laurence Perreault-Levasseur is the Canada Research Chair in Computational Cosmology and Artificial Intelligence. She is an assistant professor at Université de Montréal and an associate academic member of Mila – Quebec Artificial Intelligence Institute. Perreault-Levasseur’s research focuses on the development and application of machine learning methods to cosmology.
She is also a Visiting Scholar at the Flatiron Institute in New York City. Prior to that, she was a research fellow at their Center for Computational Astrophysics, and a KIPAC postdoctoral fellow at Stanford University.
For her PhD degree at the University of Cambridge, she worked on applications of open effective field theory methods to the formalism of inflation. She completed her BSc and MSc degrees at McGill University.