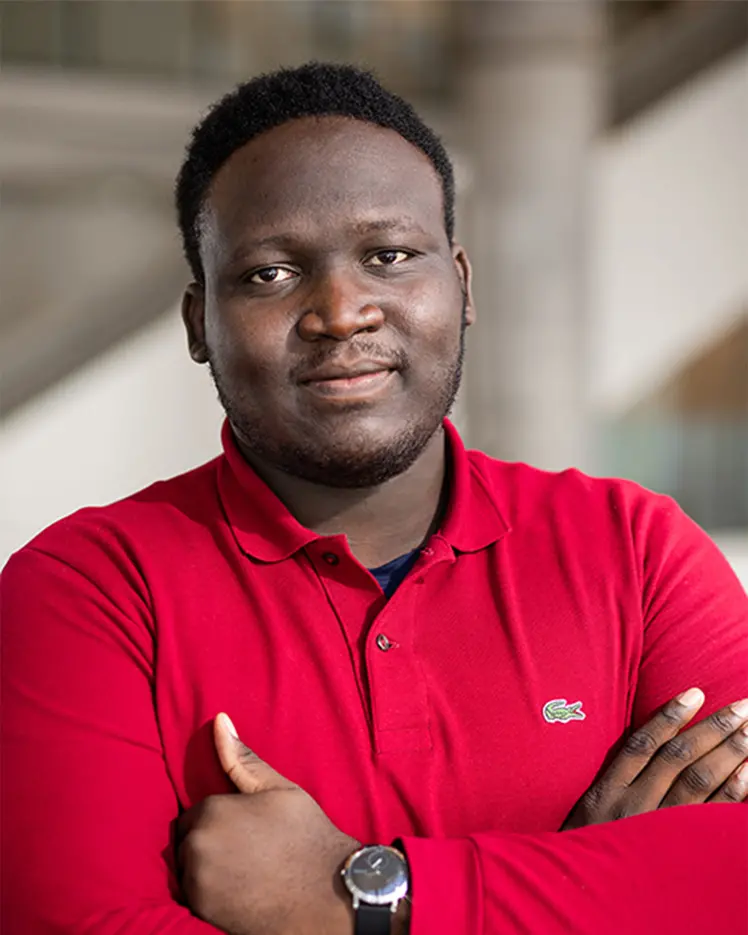
Ulrich Aivodji
Biography
Ulrich Aïvodji is an assistant professor of computer science in the Software and Information Technology Engineering Department of the École de technologie supérieure (ÉTS) in Montréal. He also leads the Trustworthy Information Systems Lab (TISL).
Aïvodji’s research areas are computer security, data privacy, optimization and machine learning. His current research focuses on several aspects of trustworthy machine learning, such as fairness, privacy-preserving machine learning and explainability.
Before his current position, he was a postdoctoral researcher at Université du Québec à Montréal, where he worked with Sébastien Gambs on machine learning ethics and privacy.
He earned his PhD in computer science from Université Paul-Sabatier (Toulouse) under the supervision of Marie-José Huguet and Marc-Olivier Killijian. He was affiliated with two research groups at the Systems Analysis and Architecture Laboratory–CNRS, one on dependable computing, fault tolerance and operations research, and another on combinatorial optimization and constraints.