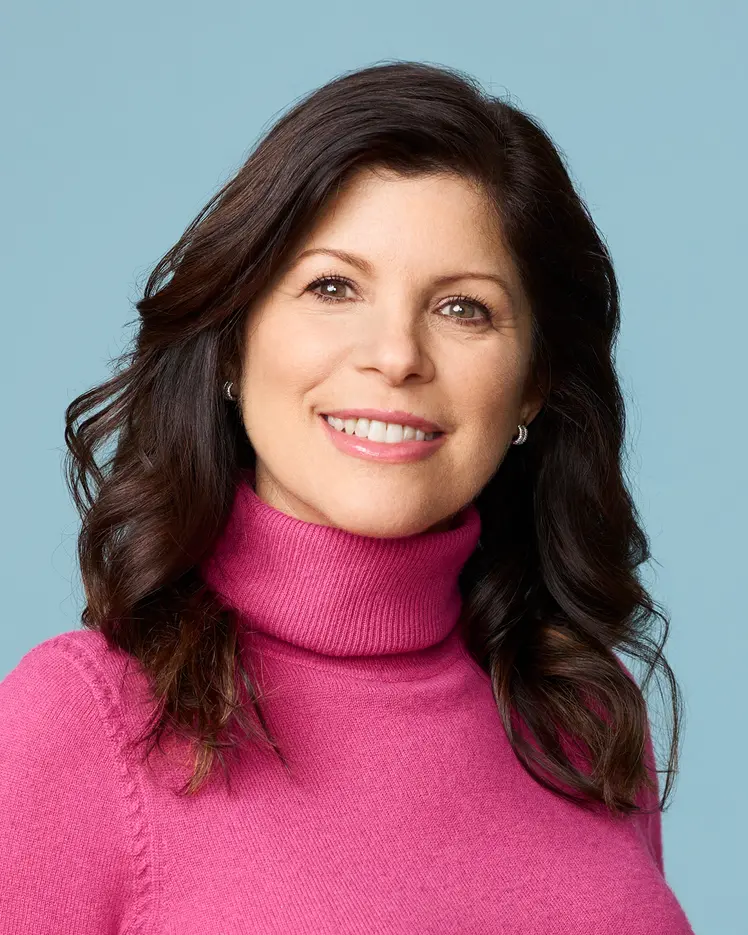
Tal Arbel
Biography
Tal Arbel is a professor in the Department of Electrical and Computer Engineering at McGill University, where she is the director of the Probabilistic Vision Group and Medical Imaging Lab in the Centre for Intelligent Machines.
She is also a Canada CIFAR AI Chair, an associate academic member of Mila – Quebec Artificial Intelligence Institute and an associate member of the Goodman Cancer Research Centre.
Arbel’s research focuses on the development of probabilistic deep learning methods in computer vision and medical image analysis for a wide range of real-world applications, with a focus on neurological diseases.
She is a recipient of the 2019 McGill Engineering Christophe Pierre Research Award and a Fellow of the Canadian Academy of Engineering. She regularly serves on the organizing team of major international conferences in computer vision and in medical image analysis (e.g. MICCAI, MIDL, ICCV, CVPR). She is currently the Editor-in-Chief and co-founder of the arXiv overlay journal: Machine Learning for Biomedical Imaging (MELBA).