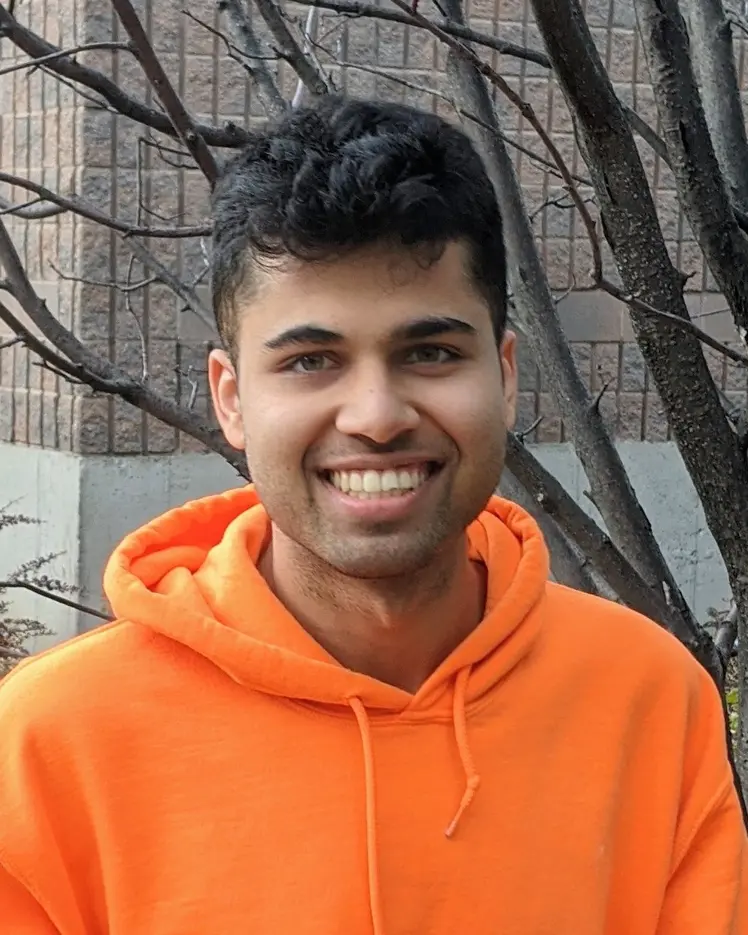
Rishabh Agarwal
Biography
I am a research scientist in the Google DeepMind Team in Montréal. I am also an Adjunct Professor at McGill University and an Associate Industry Member at Mila - Quebec Artificial Intelligence Institute. I finished my PhD at Mila under the guidance of Aaron Courville and Marc Bellemare. Previously, I spent a year at Geoffrey Hinton's amazing team in Google Brain, Toronto. Earlier, I graduated in Computer Science and Engineering from IIT Bombay.
My research work mainly revolves around language models and deep reinforcement learning (RL), and includes an outstanding paper award at NeurIPS.