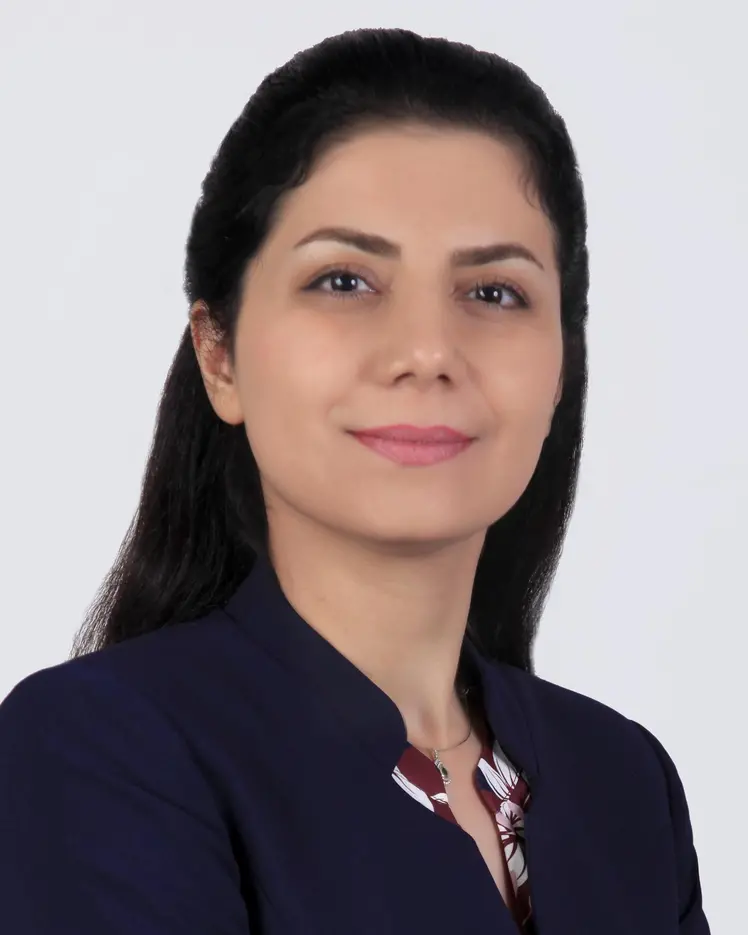
Narges Armanfard
Biography
Narges Armanfard (PhD, PEng) is the Founder and Principal Investigator of McGill University’s iSMART Lab. She's a tenure-track Assistant professor in the Department of Electrical and Computer Engineering at McGill and an Associate Academic member at Mila – Quebec Artificial Intelligence Institute.
Armanfard is also affiliated with McGill’s Centre for Intelligent Machines (CIM), the McGill initiative in Computational Medicine (MiCM), and the McGill Institute for Aerospace Engineering (MIAE).
Her research focuses on developing innovative algorithms for various domains, such as time-series data analysis, computer vision, reinforcement learning and representation learning for tasks like data clustering, classification and anomaly detection.
Her contributions to the field of AI have been recognized with numerous awards from a variety of institutions, including the Natural Sciences and Engineering Research Council of Canada, AgeWell, Vanier-Banting and the Fonds de recherche du Québec, as well as McMaster University, McGill University, the University of Toronto, the Canadian Institutes of Health Research and Scale AI.