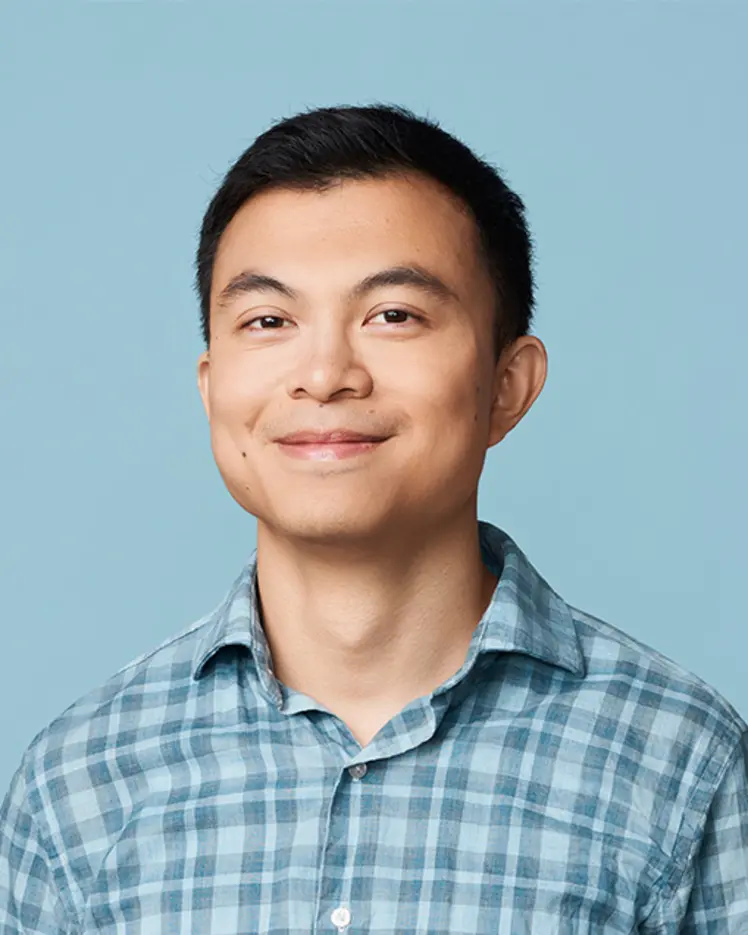
Jackie Cheung
Biography
I am an associate professor in the School of Computer Science at McGill University and a consultant researcher at Microsoft Research.
My group investigates natural language processing, an area of AI research that builds computational models of human languages, such as English or French. The goal of our research is to develop computational methods for understanding text and speech in order to generate language that is fluent and context appropriate.
In our lab, we investigate statistical machine learning techniques for analyzing and making predictions about language. Some of my current projects focus on summarizing fiction, extracting events from text, and adapting language across genres.