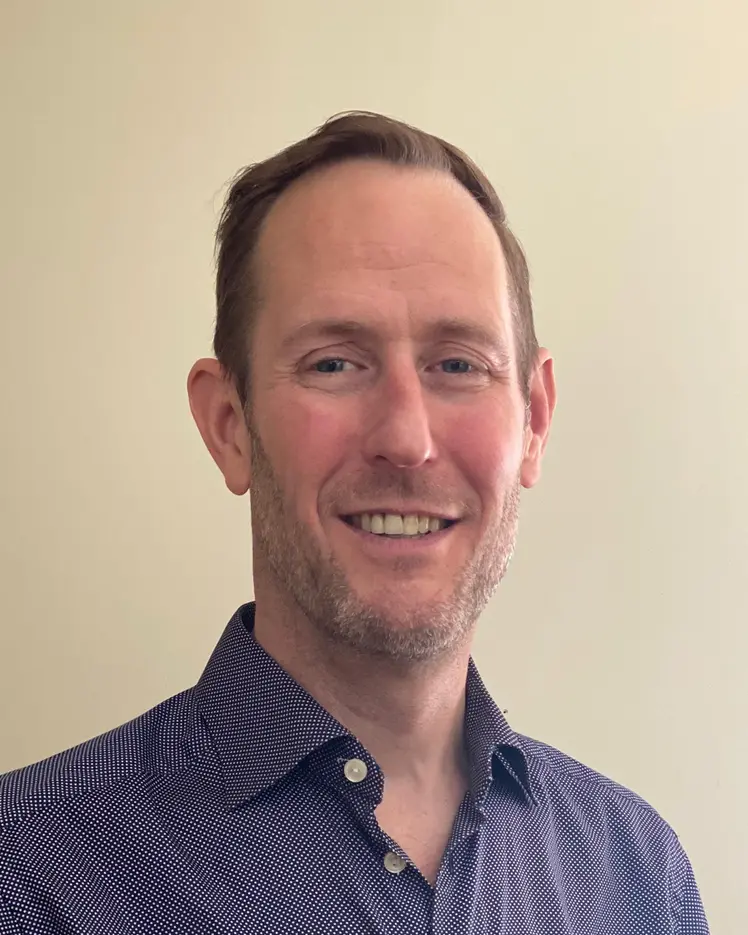
David Buckeridge
Biography
David Buckeridge is a professor at the School of Population and Global Health at McGill University, as well as chief digital health officer for the McGill University Health Centre and executive scientific director of the Public Health Agency of Canada.
A Tier 1 Canada Research Chair in Health Informatics and Data Science, Buckeridge has projected health system demand for the Canadian province of Quebec, led data management and analytics for the Canadian Immunity Task Force, and supported the World Health Organization in monitoring global immunity to SARS-CoV-2. He has an MD from Queen's University, an MSc in epidemiology from the University of Toronto and a PhD in biomedical informatics from Stanford University. He is a Fellow of the Royal College of Physicians of Canada.